What is Biometrics?
- Biometrics is a field dedicated to identifying and verifying individuals based on physiological and behavioral characteristics. This discipline leverages various technologies to compare and analyze unique personal traits to authenticate or recognize individuals.
- Biometric systems primarily operate through two main functions: verification and identification. Verification systems confirm or reject an individual’s claimed identity by comparing it to pre-stored biometric data. In contrast, identification systems search through an entire database to find a match for the queried biometric data. Both processes rely on pattern recognition techniques to ascertain the authenticity of biometric traits.
- A biometric trait, also known as a biometric, can be a physiological feature like fingerprints or iris patterns, or a behavioral characteristic such as voice or typing rhythm. These systems are integral to modern security applications, offering a reliable means of authentication by utilizing unique personal identifiers.
- In the broader context of biology and systematics, biometrics intersects with methodologies used to study and classify organisms. The field employs two main approaches: phenetic and cladistic methods. The phenetic method classifies organisms based on overall similarity, regardless of whether these similarities are derived from shared primitive traits (symplesiomorphies) or derived traits (synapomorphies). This method emphasizes the general resemblance between taxa.
- Conversely, the cladistic method, or phylogenetic approach, focuses exclusively on synapomorphies, which are shared derived characteristics. This method constructs phylogenetic trees or cladograms to illustrate the evolutionary relationships between organisms, disregarding shared primitive traits that are not useful for determining evolutionary lineage.
- Overall, biometrics combines advanced technology with biological principles to enhance identification and verification processes. By integrating biometric techniques with systematic approaches, researchers and practitioners can better understand and utilize unique personal and biological characteristics.
What is Numerical Taxonomy?
- Numerical taxonomy, also referred to as taximetrics or phenetics, represents an approach in biological classification that applies mathematical techniques to evaluate and categorize organisms based on observable traits. The fundamental aim of numerical taxonomy is to analyze and classify organisms through the quantitative assessment of similarities and differences.
- The concept of numerical taxonomy was first introduced by the French naturalist Michel Adanson in 1763. In his seminal work, “Familles des Plantes,” Adanson proposed a system that utilized various characters, such as ovary position or inflorescence type, to classify plants. This innovative approach laid the groundwork for what would later evolve into numerical taxonomy. Adanson’s method was distinguished by its use of multiple characters for classification, ensuring a more comprehensive assessment of plant similarities.
- Numerical taxonomy, or taximetrics, involves the application of mathematical procedures to numerical data representing observable characteristics of organisms. This approach does not generate new data but rather uses existing data to evaluate and categorize taxonomic groups. The technique is heavily reliant on statistical and computational methods to assess the overall similarity between organisms, facilitating the construction of taxonomic groups based on these similarities.
- In essence, numerical taxonomy seeks to classify organisms by quantitatively analyzing a broad range of traits and their variations. This method involves two key aspects: the construction of taxonomic groups based on cluster analysis, which groups organisms with similar characteristics, and the discrimination of these groups to distinguish overlapping traits and refine classifications.
- The development of numerical taxonomy gained momentum between 1957 and 1961, with significant contributions from Sneath and Sokal. They expanded upon Adansonian principles and integrated modern data-processing techniques to enhance classification methods. This evolution has led to more precise and systematic classifications, particularly in plant taxonomy.
- Therefore, numerical taxonomy, by employing mathematical and statistical methods, provides a rigorous framework for evaluating and organizing taxonomic data. It emphasizes the objective analysis of observable traits, allowing for the construction of taxonomic hierarchies based on quantitative assessments of similarity. This approach contrasts with more traditional classification methods that may rely more on subjective or qualitative assessments.
Principle of Numerical Taxonomy
The principle of numerical taxonomy, as articulated by Sneath and Sokal (1963), comprises several key tenets that guide its application in classifying and analyzing organisms. These principles emphasize a systematic and empirical approach to taxonomy, leveraging mathematical methods to ensure objectivity and accuracy.
- Empirical Science Approach:
- Numerical taxonomy should be practiced as an empirical science, meaning it relies on observable and measurable data rather than subjective opinions.
- This approach ensures that classifications are grounded in actual data rather than theoretical assumptions or subjective interpretations.
- Basis on Morphological Similarities:
- Classifications must be based on morphological similarities among organisms.
- By focusing on observable traits, numerical taxonomy seeks to create more objective and quantifiable classifications.
- Reliability with Large Number of Characters:
- Classifications derived from a large number of characters are considered more reliable.
- Using a broad range of traits reduces the potential for bias and increases the robustness of the classification system.
- Equal Weightage of Characters:
- Every character should be given equal weight in the classification process.
- This principle ensures that no single trait disproportionately influences the classification, promoting fairness and accuracy.
- Overall Similarity as a Function of Individual Similarities:
- The overall similarity between two entities is a function of their individual similarities across all the characters being compared.
- This means that a comprehensive assessment of similarity involves evaluating each trait independently and then integrating these assessments.
- Recognition of Distinct Taxa:
- Distinct taxa can be identified based on the correlation of characters, which differs among groups of organisms.
- Variations in character correlations help in distinguishing between different taxonomic groups.
- Phylogenetic Inferences from Taxonomic Structure:
- Phylogenetic relationships can be inferred from the taxonomic structure and character correlations, assuming certain evolutionary pathways and mechanisms.
- This principle allows for the reconstruction of evolutionary relationships based on the observed similarities and differences among taxa.
These tenets collectively provide a framework for numerical taxonomy that emphasizes systematic, data-driven classification, promoting greater objectivity and consistency in the study of biological diversity.
Methodology of numerical taxonomy
The methodology of numerical taxonomy involves a series of systematic steps to classify organisms based on quantifiable traits. This approach employs mathematical techniques to assess similarities and differences among operational taxonomic units (OTUs), which can be individual organisms, populations, species, or genera.
- Selection of Taxa:
- The initial step is to select the OTUs for analysis. These are the entities being classified and can vary in scope from individual organisms to higher taxonomic levels such as genera.
- Proper selection of OTUs is crucial as it defines the scope and relevance of the subsequent classification.
- Selection of Characters:
- A minimum of 60 characters is required for numerical taxonomy, though 100 characters are considered ideal for more robust classifications.
- These characters, which can be morphological, anatomical, or behavioral traits, must be described or measured. The use of a large number of characters necessitates the application of computational tools for efficient data handling.
- Coding these characters systematically is essential for accurate analysis. Each character is recorded as a presence or absence, which is crucial for the creation of the character-taxon matrix.
- Construction of Character-Taxon Matrix:
- The character-taxon matrix organizes the coded data into a tabular format, where rows represent the characters and columns represent the OTUs.
- For example, if classifying five plant species, the matrix would include data on the presence (‘1’) or absence (‘0’) of each character across these species. This matrix serves as the foundational data for subsequent analysis.
- Measurement of Phenetic Similarity:
- The phenetic similarity between each pair of OTUs is assessed by comparing their character states. This comparison calculates the overall similarity (S) and helps determine the taxonomic structure by identifying possible groups and sub-groups.
- Each character is compared across all OTUs, and the results are expressed in percentage terms. A 100% similarity (S) indicates identical taxa, while a 0% similarity denotes no resemblance at all.
- This analysis allows for the detection of taxonomic relationships and the grouping of OTUs based on their overall similarity.
Therefore, the methodology of numerical taxonomy provides a structured approach to classification by quantifying and analyzing observable traits. By using extensive character data and mathematical techniques, it ensures a more objective and systematic classification process.
Application of numerical taxonomy
The application of numerical taxonomy spans various domains of biological research, offering valuable insights and refining classification systems across different groups of organisms. This method is particularly useful in distinguishing and elucidating relationships among taxa.
- Applications in Microbiology and Zoology:
- Numerical taxonomy is extensively utilized in microbiology to differentiate between bacterial species. By analyzing various morphological and biochemical traits, taxonomists can classify and identify bacterial strains with increased precision.
- This method is also applied in zoology to classify and understand the relationships between different animal groups. For example, numerical taxonomy helps in elucidating the similarities and differences among diverse animal taxa, aiding in the development of more accurate classification systems.
- Applications in Angiosperms:
- Numerical taxonomy has been applied to several angiosperm genera, including Oryza (rice), Solanum (a genus of nightshades), and Sarcostemma (a genus of milkweeds). Studies such as those by Morishima (1969) on Oryza and Soria and Heiser (1961) on Solanum illustrate the effectiveness of this method in refining plant classifications.
- For instance, research by Hamann (1961) on the Farinosae of Engler demonstrated that numerical taxonomy could provide new insights into plant relationships and classifications.
- Validation and Revisions:
- The results obtained from numerical taxonomy often align with traditional classifications, reinforcing the validity of earlier taxonomic systems. However, this method can also reveal discrepancies. For example, in the case of Farinosae, numerical taxonomy identified some previously accepted groupings as unnatural, suggesting the need for revised classifications.
- Similarly, numerical taxonomy studies on Ononis from the Papilionaceae family confirmed the classification proposed by Sirjaev (1932), demonstrating the method’s reliability in validating existing taxonomic frameworks.
- Refining Phylogenetic Understanding:
- Numerical taxonomy has been instrumental in refining the classification of grasses. Through detailed analysis, it has been proposed that grasses may have evolved from palms. This hypothesis is supported by the observation that both groups share an ecarpellate origin of the ovary, with a greater reduction observed in grasses compared to palms.
Merits of numerical taxonomy
Numerical taxonomy offers several significant advantages over traditional taxonomic methods, enhancing the accuracy and efficiency of classification systems. The merits of numerical taxonomy are as follows:
- Comprehensive Data Utilization:
- Numerical taxonomy incorporates data from a wide range of sources, including morphology, physiology, biochemistry, amino acid sequences, and proteins. This broad data spectrum provides a more holistic view of the organisms under study compared to conventional taxonomy, which often relies on fewer sources of information.
- Accessibility for Less Experienced Taxonomists:
- The methodologies used in numerical taxonomy are structured and systematic, making them accessible to taxonomists with varying levels of expertise. This democratizes the classification process, allowing less skilled practitioners to engage effectively in taxonomic work.
- Enhanced Delimitation of Taxa:
- Numerical taxonomic methods are more sensitive and precise in differentiating between taxa. This increased sensitivity leads to the development of improved keys and classification systems, which are often more accurate than those derived from traditional methods.
- Efficient Data Handling and Application:
- Numerically coded data are easily managed and manipulated using electronic data processing systems. This capability facilitates the creation of taxonomic keys, maps, descriptions, and catalogues, enhancing the efficiency of data handling in herbaria and other taxonomic institutions.
- Improved Quality of Classification:
- By utilizing a larger number of well-described characters, numerical taxonomy improves the quality of classification systems. The comprehensive nature of the data used leads to more robust and reliable taxonomic structures.
- Revised Taxonomic Principles:
- Numerical taxonomy has prompted several fundamental changes in conventional taxonomic principles. It has refined classification systems and contributed to the evolution of new methodologies, thereby advancing the field of taxonomy.
- Reinterpretation of Biological Concepts:
- The application of numerical taxonomy has led to the re-evaluation and reinterpretation of various biological concepts. This approach has provided new insights into the relationships and classifications of organisms, influencing the broader understanding of taxonomy.
Demerits of numerical taxonomy
Numerical taxonomy, while offering numerous benefits, also presents several limitations that can affect its application and effectiveness. The demerits of numerical taxonomy are outlined below:
- Dependence on Adequate Character Selection:
- Numerical taxonomy relies heavily on machine algorithms for classification. Therefore, if the chosen characters for comparison are inadequate or poorly selected, the results may be unreliable. This dependency on accurate character selection is critical, as the effectiveness of the method hinges on the quality of the input data.
- Requirement for a Large Number of Characters:
- The methodology typically requires a large number of characters—ranging from 100 to 400—to produce satisfactory results. This poses a challenge for taxonomists who traditionally work with fewer characters. The need for extensive character data can complicate the classification process and limit the method’s applicability to organisms for which comprehensive data are available.
- Comparability with Traditional Taxonomic Methods:
- Studies, such as those by Stearn (1968), have indicated that the results obtained from numerical taxonomy using mechanical means are not always superior to those achieved through traditional taxonomic practices. The validity of groupings produced by computer-aided methods often parallels those made by experienced taxonomists, suggesting that numerical methods may not always provide significant advantages over conventional approaches.
- Neglect of Phylogenetic Relationships:
- Numerical taxonomy generally focuses on observable similarities and differences without considering the evolutionary relationships or phylogeny of the organisms being classified. This lack of emphasis on phylogeny means that the method may overlook important evolutionary connections, potentially leading to classifications that do not fully reflect the evolutionary history of the taxa.
What is Cladistic Character?
Cladistic characters are fundamental components in the field of cladistics, a method of classification that aims to reconstruct the evolutionary history of organisms. These characters are crucial for determining the branching sequences in the evolutionary tree, known as cladograms, and for establishing relationships among different taxa.
Types of Cladistic Characters:
- Derived vs. Primitive Characters:
- Derived Characters: Also referred to as apomorphic or specialized characters, these are traits that have evolved from an ancestral state. Derived characters are significant in cladistics because they help to identify evolutionary branches or clades. For example, feathers in birds are derived characters that distinguish them from other reptiles.
- Primitive Characters: Known as plesiomorphic or generalized characters, these traits are inherited from a common ancestor and have not changed significantly over evolutionary time. Primitive characters are less useful in distinguishing between closely related taxa but provide context for understanding the ancestral state.
- Synapomorphies and Symplesiomorphies:
- Synapomorphies: These are shared derived characters among a group of taxa. Synapomorphies are crucial for defining clades and establishing their evolutionary relationships. For instance, the presence of a mammary gland is a synapomorphy for mammals.
- Symplesiomorphies: These are shared primitive characters among taxa. While they indicate common ancestry, they do not provide information on the evolutionary relationships between the taxa. For example, having a backbone is a symplesiomorphy shared by all vertebrates.
Cladistic Terminology:
- Plesiotypic vs. Apotypic: Plesiotypic refers to primitive traits, while apotypic denotes derived traits. These terms, used by cladists such as Wiley (1981) and Wagner (1983), help in distinguishing between evolutionary innovations and ancestral features.
- General vs. Unique: General characters are widespread among different taxa, whereas unique characters are specific to a particular group or lineage.
Types of Diagrams:
- Dendrogram: A branching diagram that illustrates the degree of relationship between different taxa. It depicts how closely or distantly related various organisms are.
- Phenogram: A graphical representation of phenetic relationships, based on overall similarity rather than evolutionary lineage.
- Cladogram: A diagram specifically depicting cladistic relationships. It shows the branching patterns of evolution based on shared derived characters and is used to reconstruct the evolutionary history of organisms.
What is Cladogram?
A cladogram is a diagram employed in cladistics to depict the relationships among various organisms. Unlike evolutionary trees, cladograms do not illustrate direct ancestral-descendant relationships or the amount of evolutionary change. Instead, they provide a framework for understanding the relative relationships based on shared characteristics.
Key Features of Cladograms:
- Branching Structure:
- Representation of Relationships: Cladograms use lines that branch off from a central axis, leading to different clades. A clade is a group consisting of an ancestor and all its descendants. These branching points, called nodes, represent hypothetical common ancestors.
- Lineage Tracking: The branches of a cladogram can be traced back to their origin, showing the lineage from a common ancestor to the present-day taxa.
- Hypothetical Ancestors:
- Inference of Traits: The nodes in a cladogram denote hypothetical ancestors that share traits with the terminal taxa (the end points of the branches). These traits help infer the evolutionary order of various features and adaptations.
- Evolutionary Narratives: Although cladograms do not indicate the actual amount of evolutionary change, they offer insights into the evolutionary relationships and the sequence of trait development.
- Data Utilization:
- Morphological Data: Traditionally, cladograms were constructed using morphological data, which involves physical characteristics and traits.
- Modern Techniques: With advancements in technology, DNA and RNA sequencing data are now frequently used in conjunction with morphological data to construct more accurate and detailed cladograms.
- Shapes and Variations:
- Diverse Forms: Cladograms can vary in shape but universally feature branching lines. These shapes reflect different patterns of relationships and are used to highlight evolutionary connections.
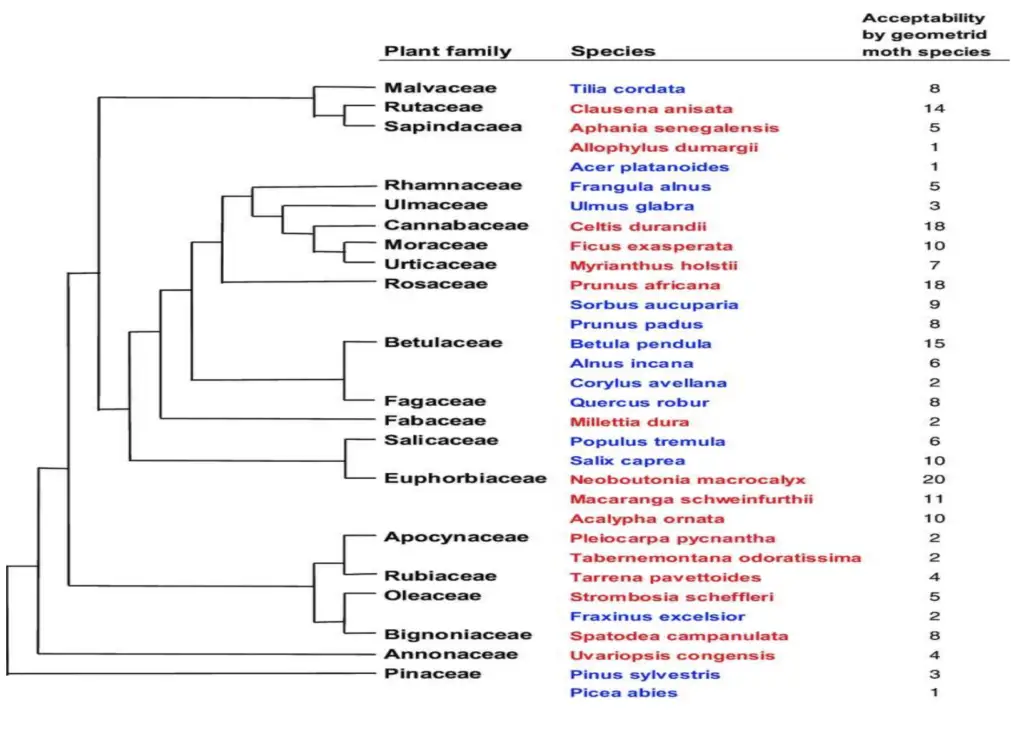
What is Phenograms?
A phenogram is a type of dendrogram used to represent the relationships among organisms based on phenetic data. Unlike cladograms, which focus on evolutionary relationships, phenograms are constructed using similarity measures derived from a range of phenetic features.
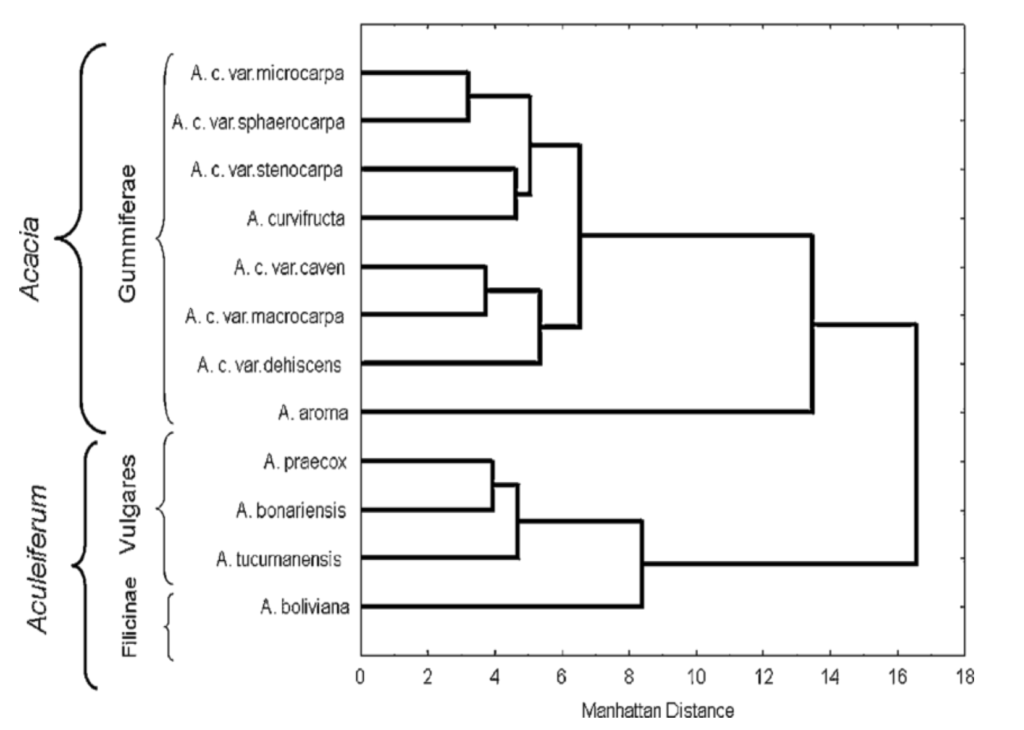
Key Features of Phenograms:
- Phenetic Basis:
- Data Source: Phenograms are constructed from phenetic data, which involves numerical analysis of observable characteristics across organisms. This method emphasizes overall similarity rather than evolutionary lineage.
- Phenon Lines: In a phenogram, lines called phenon lines are drawn at right angles to the main branching structure. These lines illustrate the percentage similarity of phenetic features between different organisms.
- Construction Process:
- Character Data: The construction of a phenogram relies on a comprehensive set of character data collected from various sources. This includes numerous attributes and traits of the organisms under study.
- Cluster Analysis: Similarity between taxa is calculated, and a diagram is created through cluster analysis. This process groups organisms based on their phenetic similarity, resulting in a hierarchical classification.
- Hierarchical Classification:
- Similarity Assessment: Phenograms enable hierarchical classification by determining the degree of similarity between taxa. This classification is organized into ranks, reflecting the relative closeness of different organisms based on their phenetic features.
- Broad Utility: Due to the inclusion of a large number of characters, phenograms provide a detailed representation of the relationships among organisms, making them valuable for extensive comparative studies.
- Applications and Utility:
- Comprehensive Representation: Phenograms are useful for representing the relationships among organisms based on a broad array of characteristics. This approach allows for a more inclusive comparison of phenotypic traits.
- Versatility: The ability to incorporate a large dataset and perform detailed cluster analysis makes phenograms an effective tool for examining similarities and constructing detailed hierarchical classifications.
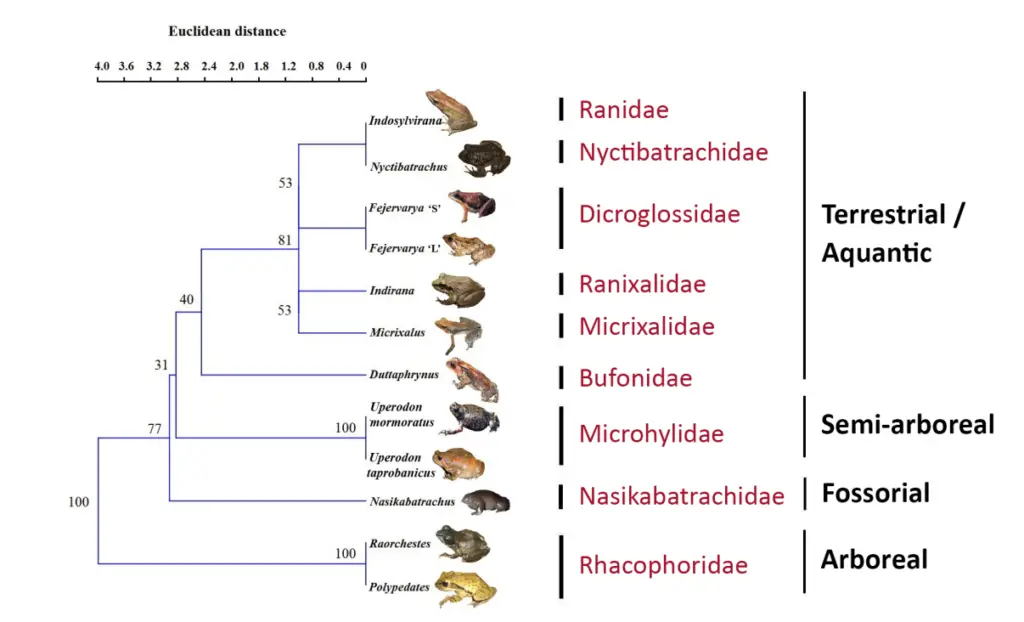
Construction of Taxonomic Groups
The construction of taxonomic groups involves several systematic steps that aid in organizing and classifying organisms based on their characteristics. This process is fundamental in taxonomy and aims to categorize organisms into meaningful groups that reflect their evolutionary relationships. The primary steps involved in this process are as follows:
- Operational Taxonomic Units (OTUs):
- Definition and Scope: OTUs represent the basic units in numerical taxonomy. They can be individuals, species, genera, families, orders, or classes. Unlike formal taxonomic units, OTUs are operational and are defined for the specific purpose of comparison within a study. This flexibility allows taxonomists to work with various levels of biological organization.
- Representation: For OTUs beyond the individual level, such as genera or families, it is essential to include diverse representatives. For instance, when comparing genera, multiple species should be included to ensure a comprehensive analysis. Similarly, comparisons at the family level should involve different genera to capture the full spectrum of diversity.
- Application: OTUs are utilized to standardize comparisons across different taxonomic levels, providing a consistent framework for classification.
- Unit Characters:
- Definition: Unit characters are the specific traits used in numerical taxonomy to differentiate between OTUs. According to Sokal and Sneath (1963), a unit character is a taxonomic trait with two or more states that cannot be further subdivided except through changes in coding methods. These characters are primarily phenotypic, such as the presence or absence of specific structures.
- Types of Unit Characters:
- Binary Characters: These characters exist in two states, such as the presence or absence of trichomes. They are coded simply as +/– or 1/0. If a character is not present, it is recorded as NC (no comparison).
- Multistate Characters: These characters have more than two possible states and are coded numerically. They are further divided into:
- Qualitative Multistate Characters: These involve distinct categories without a specific order, such as flower color with states like red, white, and yellow.
- Quantitative Multistate Characters: These involve continuous measurements, such as leaf length or plant height, which can be quantified in various increments.
- Estimation of Resemblances:
- Measures of Similarity and Dissimilarity: To construct taxonomic groups, the resemblance between OTUs is assessed using similarity or dissimilarity measures. Similarity is measured as the percentage of shared characters, while dissimilarity reflects the percentage of characters in which OTUs differ. These metrics help quantify how closely related or distinct different OTUs are.
- Application: By calculating these measures, taxonomists can evaluate the degree of relatedness between different groups, guiding the construction of taxonomic classifications.
- Cluster Analysis:
- Purpose and Process: Cluster analysis is a multivariate statistical technique used to group OTUs based on their similarity. The goal is to identify clusters of organisms that share similar traits, which can then be used to understand their relationships.
- Characteristics:
- Mathematical Formulation: Cluster analysis relies on mathematical models to measure similarity among organisms. These models use various metrics to quantify how closely related different operational taxonomic units (OTUs) are.Types of Clusters:
- Numerical Clusters: These clusters are based on quantitative measures of similarity.
- Statistical Clusters: These clusters are defined using statistical methods to analyze data patterns.
- Conceptual Clusters: These are based on theoretical constructs that describe relationships among data points.
- Approaches to Cluster Analysis:
- Agglomerative vs. Divisive:
- Agglomerative Methods: This approach begins with individual taxa and progressively merges them into pairs or groups to maximize similarity at each step. The process continues until all taxa are clustered into a single group or until a predefined number of clusters is achieved.
- Divisive Methods: This method starts with a single group and splits it into smaller groups to maximize differences between the groups formed. This approach focuses on separating clusters to highlight significant disparities.
- Overlapping vs. Disjoint Clusters:
- Overlapping Clusters: These clusters allow for the possibility that an organism may belong to more than one group based on shared characteristics.
- Disjoint Clusters: These clusters do not overlap; each organism is assigned to a single cluster only.
- Incremental vs. Non-Incremental:
- Incremental Methods: These methods build clusters step-by-step, adding elements incrementally based on similarity measures.
- Non-Incremental Methods: These methods consider all data simultaneously to form clusters without incremental steps.
- Flat vs. Hierarchical Clusters:
- Flat Clusters: These clusters are formed in a single level without any nested grouping.
- Hierarchical Clusters: These involve multiple levels of grouping, where clusters are nested within other clusters.
- Agglomerative vs. Divisive:
- Clustering Methods:
- Monothetic System: This approach evaluates one attribute at a time to form clusters. While straightforward, it often results in artificial clustering since it does not consider the full range of attributes simultaneously.
- Holistic System: This method examines all attributes of organisms simultaneously, leading to more natural and representative groupings.
- Phenetic Clustering Methods: Historically, phenetic methods were used for clustering based on overall similarity. However, these methods have largely been replaced by cladistic approaches that relate data to evolutionary history. Phenetic clustering methods are less common in contemporary taxonomy as they often produce clusters that differ significantly from those derived from cladistic methods.
- Techniques in Cluster Analysis:
- Elementary Cluster Analysis: This technique involves basic methods to group OTUs based on similarity measures.
- Linkage Methods:
- Single Linkage: Groups are formed by linking pairs with the smallest distance, potentially leading to long, stringy clusters.
- Complete Linkage: Clusters are formed by considering the maximum distance between members, which can result in compact, well-defined clusters.
- Average Linkage: This method averages the distances between all members of the clusters, balancing the formation of clusters.
- Central or Nodal Clustering: This technique focuses on central points or nodes to form clusters based on proximity to these central figures.
Difference Between Phenograms and cladograms
Phenograms and cladograms are both graphical representations used to visualize relationships among organisms, but they serve different purposes and are based on distinct principles. Understanding these differences is crucial for interpreting the data they represent. Here is a detailed comparison of the two:
- Definition and Purpose:
- Cladogram:
- Definition: A cladogram is a diagram that represents the hypothetical evolutionary relationships among a group of organisms. It is used to depict the branching patterns of evolutionary lineages.
- Purpose: Cladograms illustrate the relative relationships between taxa but do not indicate the actual evolutionary history or distances between them.
- Phenogram:
- Definition: A phenogram is a graphical representation of the phenetic relationships among organisms based on observed similarities and differences in their characteristics.
- Purpose: Phenograms are used to show the overall similarity among taxa, grouping organisms based on the degree of phenotypic resemblance.
- Cladogram:
- Representation of Evolutionary Relationships:
- Cladogram:
- Representation: Cladograms are not intended to represent actual evolutionary history or phylogeny. They are based on the principle of shared derived characteristics (synapomorphies) and show the relationships as a branching diagram.
- Evolutionary Relationships: They do not depict evolutionary distances or the amount of time that has passed since the divergence of taxa. Instead, they provide a framework for understanding the branching pattern of evolutionary history.
- Phenogram:
- Representation: Phenograms represent the overall similarity or dissimilarity among taxa, with the length of branches often corresponding to the degree of similarity.
- Evolutionary Relationships: While phenograms do not explicitly show evolutionary relationships, the length of the branches can provide an indication of the similarity or dissimilarity between taxa, rather than the actual evolutionary time.
- Cladogram:
- Branch Length and Interpretation:
- Cladogram:
- Branch Length: In a cladogram, branches are drawn with equal length. The length of the branches does not convey any information about the evolutionary distance or time between taxa.
- Interpretation: The focus is on the branching order and the shared characteristics among taxa, rather than the amount of evolutionary change.
- Phenogram:
- Branch Length: Branches in a phenogram vary in length to represent the degree of phenotypic similarity or dissimilarity. Longer branches typically indicate greater differences.
- Interpretation: The length of the branches provides information about the amount of observed differences between organisms, though it does not represent evolutionary time directly.
- Cladogram:
- Representation of Evolutionary Time:
- Cladogram:
- Evolutionary Time: Cladograms do not show the amount of evolutionary time that separates taxa. The focus is purely on the relationships and branching order.
- Phenogram:
- Evolutionary Time: Phenograms do not directly represent evolutionary time either; they focus on the similarity of characteristics rather than temporal changes. However, in some cases, the length of the branches can suggest the degree of phenotypic change.
- Cladogram:
Aspect | Cladogram | Phenogram |
---|---|---|
Definition | Represents hypothetical evolutionary relationships among organisms. | Represents phenetic relationships based on observed similarities and differences. |
Purpose | Illustrates branching patterns of evolutionary lineages. | Shows overall similarity among taxa, grouping based on phenotypic resemblance. |
Evolutionary Relationships | Does not represent actual evolutionary history or distances. | Does not explicitly show evolutionary relationships; focuses on similarity or dissimilarity. |
Branch Length | Branches are drawn with equal length; no information on evolutionary distance. | Branch lengths vary to indicate degree of phenotypic similarity or dissimilarity. |
Representation of Evolutionary Time | Does not indicate evolutionary time or amount of change. | Does not directly represent evolutionary time; branch length can suggest degree of phenotypic change. |
References
- S.R., Chandramouli & Dutta, Sushil. (2015). Comparative osteology of anuran genera in the Western Ghats, Peninsular India. Alytes. 32. 67-81.
- http://courseware.cutm.ac.in/wp-content/uploads/2020/05/Numerical-Taxonomy-OTUs.pdf
- https://www.deshbandhucollege.ac.in/pdf/resources/1586455931_Z(H)-II-GE-Biometrics-2.pdf
- https://www.hcpgcollege.edu.in/sites/default/files/MODERNS%20TRENDS%20IN%20PLANT%20TAXONOMY-15-03-2023.pdf
- https://library.dbca.wa.gov.au/FullTextFiles/600365.pdf
- https://eurekamag.com/research/004/840/004840987.php?srsltid=AfmBOoq8cazpzjKnrhQgZxuWz7WaazPMsv12k3P7sVaYHmmANt8zH_Un
- https://www.slideshare.net/slideshow/numerical-taxonomy-biometricspdf/258578426