AQA GCSE Biology 16 Views 1 Answers
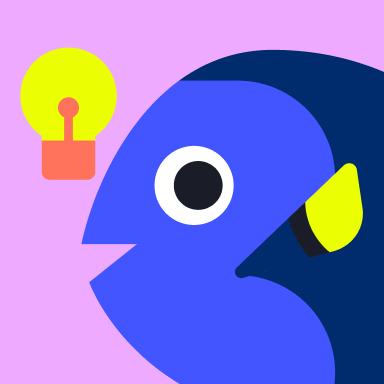
Sourav Pan🥇 GoldNovember 11, 2024
What role do scatter diagrams play in identifying correlations between two variables in disease data?
What role do scatter diagrams play in identifying correlations between two variables in disease data?
Please login to save the post
Please login to submit an answer.
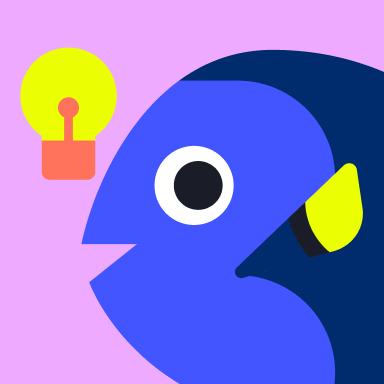
Sourav Pan🥇 GoldMay 15, 2025
Scatter diagrams, also known as scatter plots, play a crucial role in identifying correlations between two variables in disease data. Here’s how they function and their significance in epidemiological analysis:
Purpose of Scatter Diagrams
- Visual Representation of Relationships:
- Scatter diagrams graphically display the relationship between two quantitative variables, with one variable plotted along the x-axis (independent variable) and the other along the y-axis (dependent variable). This visual representation helps to quickly identify any correlation that may exist between the two variables being studied.
- Correlation Identification:
- By plotting data points, scatter plots allow researchers to observe patterns that indicate the strength and direction of a relationship. For example:
- Positive Correlation: As one variable increases, the other also increases, represented by points that trend upward from left to right.
- Negative Correlation: As one variable increases, the other decreases, indicated by points trending downward.
- No Correlation: Points are scattered randomly without any discernible pattern, suggesting no relationship between the variables.
- By plotting data points, scatter plots allow researchers to observe patterns that indicate the strength and direction of a relationship. For example:
Analyzing Disease Data
- Assessing Variables:
- In disease research, scatter diagrams can be used to explore potential relationships between various factors, such as:
- The relationship between environmental exposures (e.g., pollution levels) and disease incidence rates (e.g., respiratory diseases).
- The correlation between lifestyle factors (e.g., physical activity) and health outcomes (e.g., obesity rates).
- In disease research, scatter diagrams can be used to explore potential relationships between various factors, such as:
- Strength and Shape of Relationships:
- Scatter plots help assess not just whether a correlation exists but also its strength (strong, moderate, weak) and shape (linear or nonlinear). This analysis can inform further statistical testing or hypothesis generation regarding causal relationships .
- Statistical Testing:
- While scatter plots visually suggest correlations, statistical tests like Pearson’s correlation coefficient can quantify the strength of the relationship. This is essential for determining whether observed correlations are statistically significant.
Challenges and Considerations
- Causation vs. Correlation:
- It is important to note that scatter plots indicate correlation but do not imply causation. Researchers must be cautious not to infer that changes in one variable directly cause changes in another without further investigation .
- Overplotting:
- In datasets with a large number of observations, points may overlap (overplotting), making it difficult to discern relationships. Techniques such as transparency adjustments or using smaller point sizes can help mitigate this issue .
- Outliers:
- Scatter diagrams can reveal outliers—data points that deviate significantly from the overall pattern—which may indicate unique cases or errors in data collection that warrant further investigation
0
0 likes
- Share on Facebook
- Share on Twitter
- Share on LinkedIn