Genetics 8 Views 1 Answers
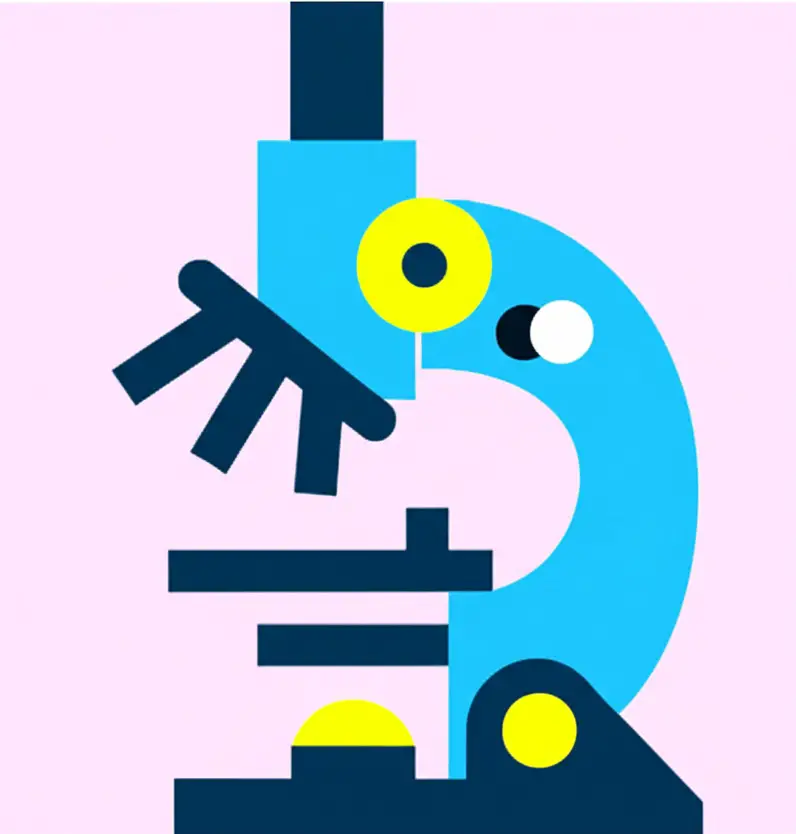
Sourav Pan🥇 GoldSeptember 10, 2024
what is a chi-square test?
what is a chi-square test
Please login to save the post
Please login to submit an answer.
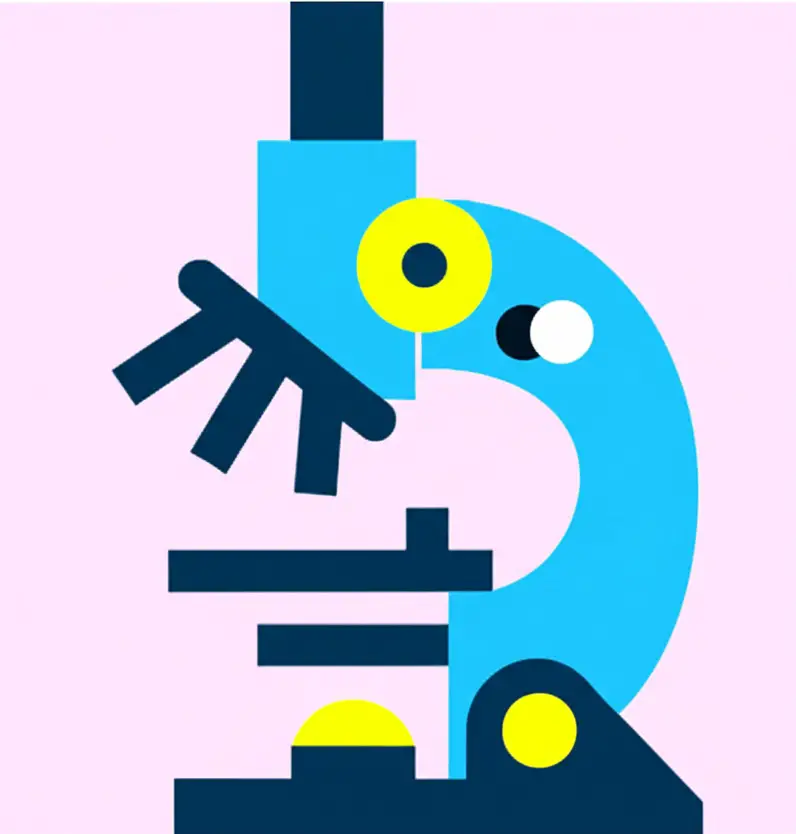
Sourav Pan🥇 GoldMay 15, 2025
A Chi-Square test is a statistical method used to evaluate the relationship between categorical variables or to assess how well observed data fit a theoretical distribution. It is widely used in hypothesis testing to determine if there are significant differences between expected and observed frequencies in categorical data. Here’s a detailed explanation of what a Chi-Square test involves:
Purpose
- Test of Independence:
- Objective: To determine if there is a significant association between two categorical variables.
- Example: Assessing whether gender is related to voting preference (e.g., whether male and female voters show different preferences for candidates).
- Goodness of Fit Test:
- Objective: To assess how well observed data match an expected distribution.
- Example: Testing whether a die is fair by comparing the observed frequencies of each face to the expected frequencies if each face had an equal chance of appearing.
- Test of Homogeneity:
- Objective: To compare the distribution of a categorical variable across different populations or groups to see if they are similar.
- Example: Comparing the distribution of preference for a product across different regions to see if it is consistent.
How It Works
- Calculate Expected Frequencies:
- For Independence and Homogeneity Tests: Use the marginal totals of the contingency table to compute the expected frequency for each cell in the table.
- For Goodness of Fit Test: Use the theoretical distribution to calculate the expected frequencies.
- Compute the Chi-Square Statistic:
- Formula: chi^2 = sum frac{(O_{ij} - E_{ij})^2}{E_{ij}}
-
-
- where OiO_iOi represents the observed frequency in each category, and EiE_iEi represents the expected frequency for that category.
- Procedure: Sum the squared differences between observed and expected frequencies, divided by the expected frequencies.
- Determine Significance:
- Compare to Critical Value: Compare the Chi-Square statistic to a critical value from the Chi-Square distribution table, based on the desired level of significance (alpha) and degrees of freedom.
- Calculate p-Value: Alternatively, compute the p-value to assess the significance of the Chi-Square statistic.
Assumptions
- Independence: The observations should be independent of each other.
- Sample Size: Typically, expected frequencies in each cell should be 5 or more to ensure the validity of the Chi-Square approximation.
Applications
- Social Sciences: Analyzing survey data to identify associations between demographic factors and opinions.
- Biology: Testing genetic data to see if observed allele frequencies fit expected Mendelian ratios.
- Marketing: Evaluating consumer preferences across different market segments.
-
0
0 likes
- Share on Facebook
- Share on Twitter
- Share on LinkedIn