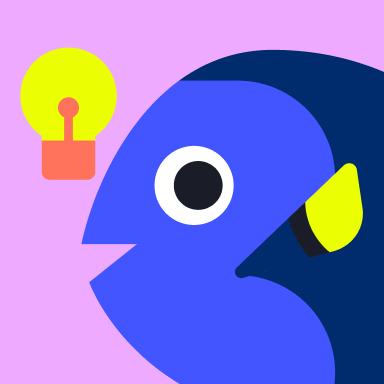
Sourav Pan
Transcript
What is a P-Value?
A p-value is the probability of obtaining results at least as extreme as those observed, if the null hypothesis were true.
To understand p-values, let’s visualize a normal distribution representing test statistics under a null hypothesis.
If we observe a test statistic beyond a critical value, we enter a region of low probability under the null hypothesis.
The p-value represents the probability of observing such extreme results if the null hypothesis were true.
Let’s clarify some key points about p-values.
P-values measure the strength of evidence against the null hypothesis.
Smaller p-values indicate stronger evidence against the null hypothesis.
Important to note: a p-value is NOT the probability that the null hypothesis is true.
And it is NOT the probability that the results occurred by chance.
Now, let’s briefly discuss statistical significance.
Typically, a threshold of p less than 0.05 is used to determine significance.
Results with p-values below this threshold are considered ‘statistically significant’.
However, this doesn’t necessarily mean the effect is large or important in a practical sense.
Understanding what a p-value represents is essential for correctly interpreting statistical results.
Now let’s focus on interpreting p-values correctly.
P-values range from zero to one and serve as a universal language for interpreting statistical test results.
The most common threshold used is 0.05. Results with p-values less than 0.05 are typically considered statistically significant.
In hypothesis testing, when the p-value is less than 0.05, we typically reject the null hypothesis, indicating a statistically significant result.
Conversely, when the p-value is greater than 0.05, we fail to reject the null hypothesis, suggesting the result is not statistically significant.
To visualize p-values, we can look at a distribution curve. The p-value represents the probability of observing a test statistic as extreme or more extreme than what we observed.
The smaller the p-value, the smaller the area in the tail of the distribution, and the stronger the evidence against the null hypothesis.
At our conventional threshold of 0.05, the critical region includes these values in the tail.
Larger p-values like 0.1 indicate weaker evidence against the null hypothesis.
Let’s look at how we can interpret different ranges of p-values.
P-values less than 0.001 provide very strong evidence against the null hypothesis.
P-values between 0.001 and 0.01 offer strong evidence.
Values between 0.01 and our typical threshold of 0.05 indicate moderate evidence.
P-values between 0.05 and 0.1 suggest weak, potentially trending evidence.
Finally, p-values of 0.1 or larger generally indicate no significant evidence against the null hypothesis.
As we interpret p-values, it’s important to keep some key points in mind.
P-values do not measure the size of an effect or tell us about practical significance. The 0.05 threshold is conventional but ultimately arbitrary. What remains consistent is that smaller p-values indicate stronger evidence against the null hypothesis.
P-values are frequently misunderstood in statistical analysis. Let’s examine three common misconceptions.
Misconception number one: P-values measure the probability that the null hypothesis is true.
This is incorrect. The p-value only tells us how likely we would observe our data, or more extreme data, if the null hypothesis were true.
The correct interpretation is that the p-value is the probability of observing our data, or more extreme data, if the null hypothesis were true.
Misconception number two: P-values indicate the size or importance of an effect.
This is incorrect. Two studies can have the same p-value, but very different effect sizes.
For example, these two studies have identical p-values of zero point zero four, but their effect sizes are dramatically different.
The correct understanding is that p-values only measure statistical significance, not practical significance or the importance of findings.
Misconception number three: P-values tell us the probability of replicating results.
This is incorrect. A statistically significant result does not guarantee the same result in future studies.
The correct understanding is that p-values naturally vary from sample to sample, and a single p-value cannot predict the outcome of future replication attempts.
To summarize, remember these key points about p-values:
P-values are just one tool in the statistical toolbox, not the final answer to scientific questions.
To find p-values for t-tests, we follow a 3-step process.
First, calculate the t-statistic from your sample data. Second, determine the appropriate degrees of freedom. And third, find the corresponding p-value using the t-distribution.
The t-statistic is calculated using this formula. It measures how many standard errors the sample mean is from the hypothesized population mean.
Next, we determine the degrees of freedom. For a one-sample t-test, the degrees of freedom equal sample size minus one. For a two-sample t-test, it’s the sum of both sample sizes minus two.
Degrees of freedom represent the number of independent values that can vary in the calculation.
Finally, we use the t-distribution to find the p-value that corresponds to our calculated t-statistic and degrees of freedom.
Let’s work through a practical example. Imagine we’re testing if a new study method improves test scores.
We have a sample of 16 students, with a mean score of 78.5 and a standard deviation of 8.1. We want to test if this is significantly higher than the previous average of 75.
Let’s calculate the t-statistic using our formula. We substitute our values: sample mean 78.5, hypothesized mean 75.0, standard deviation 8.1, and sample size 16.
We simplify step by step. The square root of 16 is 4.
Dividing 8.1 by 4 gives us 2.025.
Finally, dividing 3.5 by 2.025 gives us a t-statistic of 1.728.
The degrees of freedom equals sample size minus one, which is 15.
Now let’s find the p-value using the t-distribution with 15 degrees of freedom.
Our t-statistic is 1.728. For a one-tailed test, we’re interested in the probability of observing a t-value this extreme or more extreme in the direction of our alternative hypothesis.
Let’s compare one-tailed and two-tailed tests using our example.
In a one-tailed test, we’re only concerned with the probability in one direction. In our example, the p-value is approximately 0.052.
For a two-tailed test, we consider both directions. The p-value is approximately 0.104, which is twice the one-tailed p-value.
Let’s make a decision based on our p-values and our chosen significance level of 0.05.
For our one-tailed test, the p-value is greater than alpha, so we fail to reject the null hypothesis.
This means there is insufficient evidence that the new study method significantly improves test scores at the 0.05 significance level.
Chi-square tests are used to analyze categorical data and determine if there’s a significant relationship between variables.
The chi-square statistic compares observed values to expected values using this formula.
Let’s work through an example of a chi-square test of independence to see if music preference is related to age group.
Here’s our observed data in a contingency table. We have three age groups and four music genres, with the number of people in each category.
First, we need to calculate the expected values for each cell in our table. We multiply the row total by the column total, then divide by the grand total.
Next, we calculate the chi-square statistic by summing the squared differences between observed and expected values, divided by the expected values.
We calculate the degrees of freedom by multiplying rows minus one by columns minus one. In our example, that’s two times three, giving us six degrees of freedom.
Finally, we use a chi-square distribution table or calculator to find the p-value. With a chi-square statistic of 26.14 and 6 degrees of freedom, we get a p-value less than 0.001.
Since our p-value is less than 0.05, we reject the null hypothesis. We conclude that there is a significant relationship between age group and music preference.
To summarize, chi-square tests analyze relationships between categorical variables. We calculate expected values, find the chi-square statistic, determine degrees of freedom, and interpret the p-value to draw conclusions about our data.
Modern approaches and alternatives to p-values have emerged in response to their limitations and misuse.
In 2016, the American Statistical Association issued a statement addressing the proper use and interpretation of p-values. The statement emphasized that p-values don’t measure effect size or importance, and scientific conclusions shouldn’t be based solely on p-values. It noted that p-values can be influenced by sample size, not just effect, and proper inference requires transparency and full context.
Let’s explore alternatives and complementary approaches to p-values that can provide more nuanced information.
Confidence intervals provide a range of plausible values for the parameter of interest. Unlike p-values, they show both precision through the width of the interval and magnitude through its location. A 95% confidence interval contains the true parameter value in 95% of repeated samples.
Effect sizes provide a standardized measure of the magnitude of an effect. Common measures include Cohen’s d, odds ratios, risk differences, and correlation coefficients. Unlike p-values, effect sizes are independent of sample size and facilitate comparison across studies.
Bayesian methods incorporate prior knowledge with new evidence to produce posterior probability distributions for parameters. Unlike frequentist approaches, Bayesian statistics allows for direct probability statements about hypotheses and naturally updates beliefs as new data emerges.
Multiple comparison corrections address the inflation of Type I error when conducting many statistical tests simultaneously. Methods like Bonferroni control the family-wise error rate by dividing alpha by the number of tests. Less stringent approaches like the False Discovery Rate control the proportion of false positives among rejected null hypotheses.
Let’s explore best practices for responsible statistical analysis in the modern era. Report effect sizes alongside p-values to communicate practical significance. Provide confidence intervals for parameter estimates to show precision and magnitude. Consider Bayesian alternatives when incorporating prior knowledge is valuable. Pre-register analyses to avoid p-hacking and selective reporting. Account for multiple comparisons when conducting numerous tests. And report exact p-values rather than simply stating significance thresholds.
In conclusion, p-values remain a valuable statistical tool when used appropriately and complemented with other approaches. By combining p-values with effect sizes, confidence intervals, and other methods, researchers can provide a more complete and nuanced statistical story that advances scientific understanding.
Related Videos
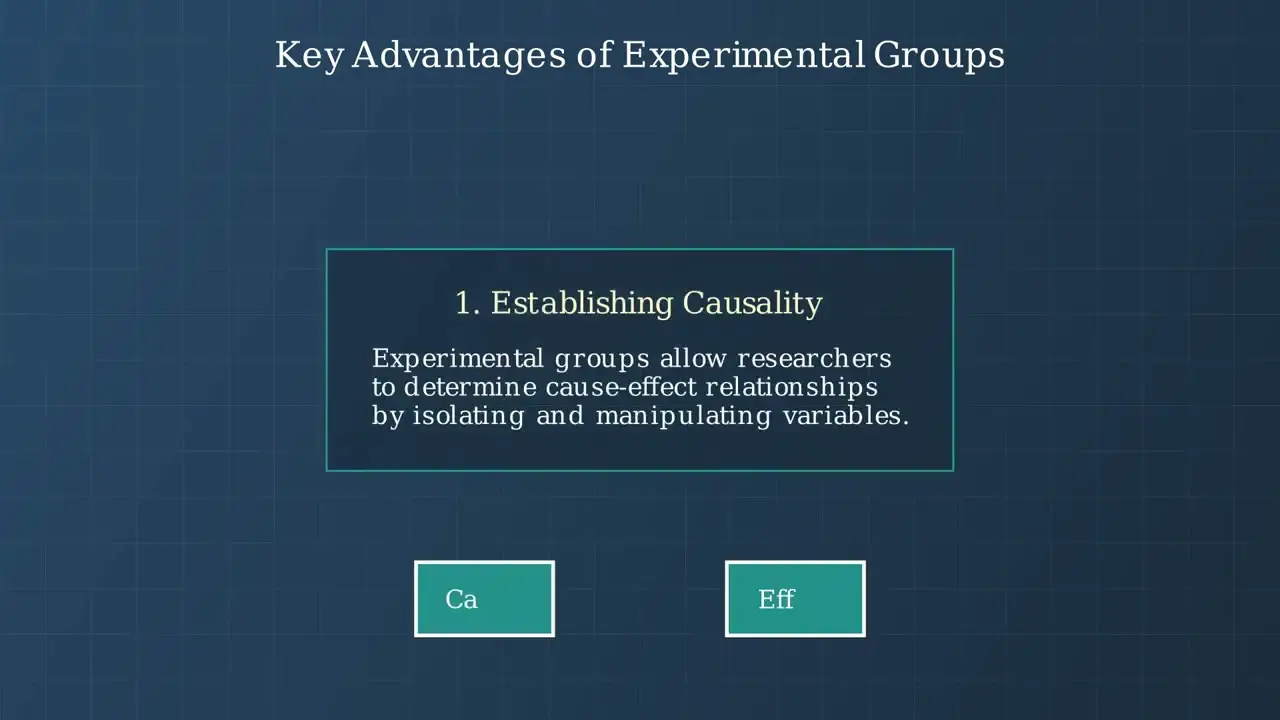
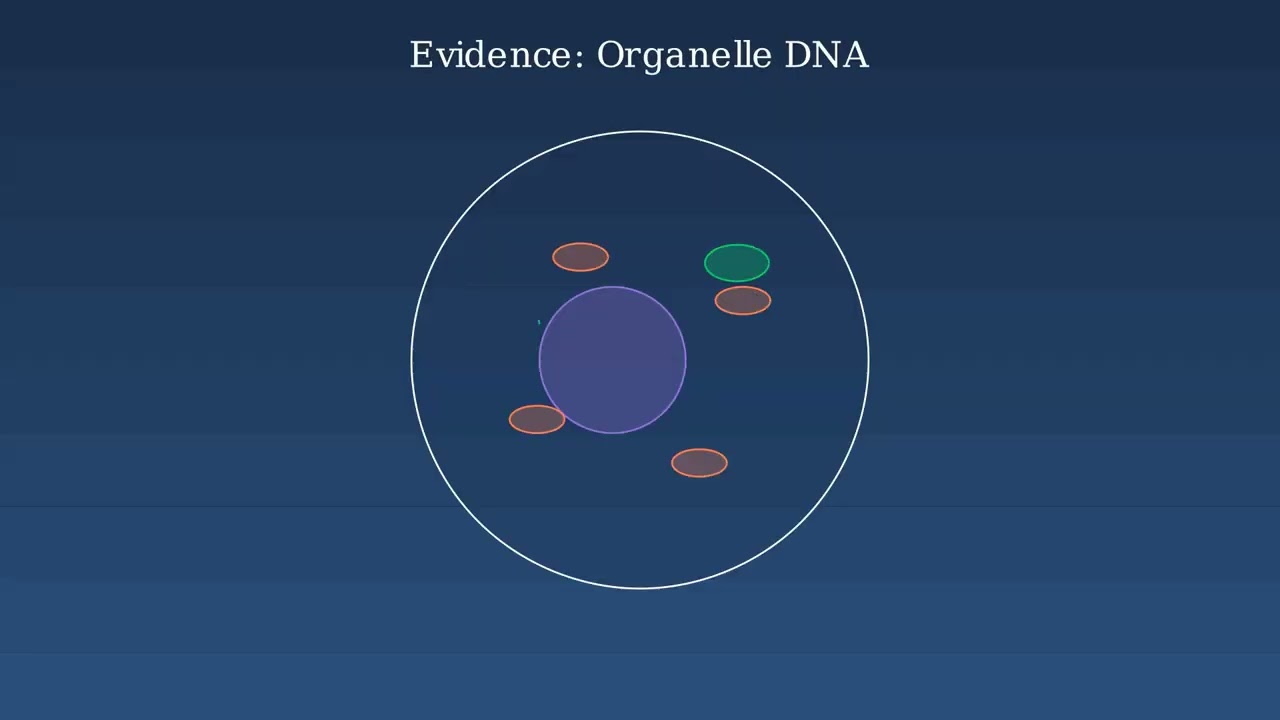
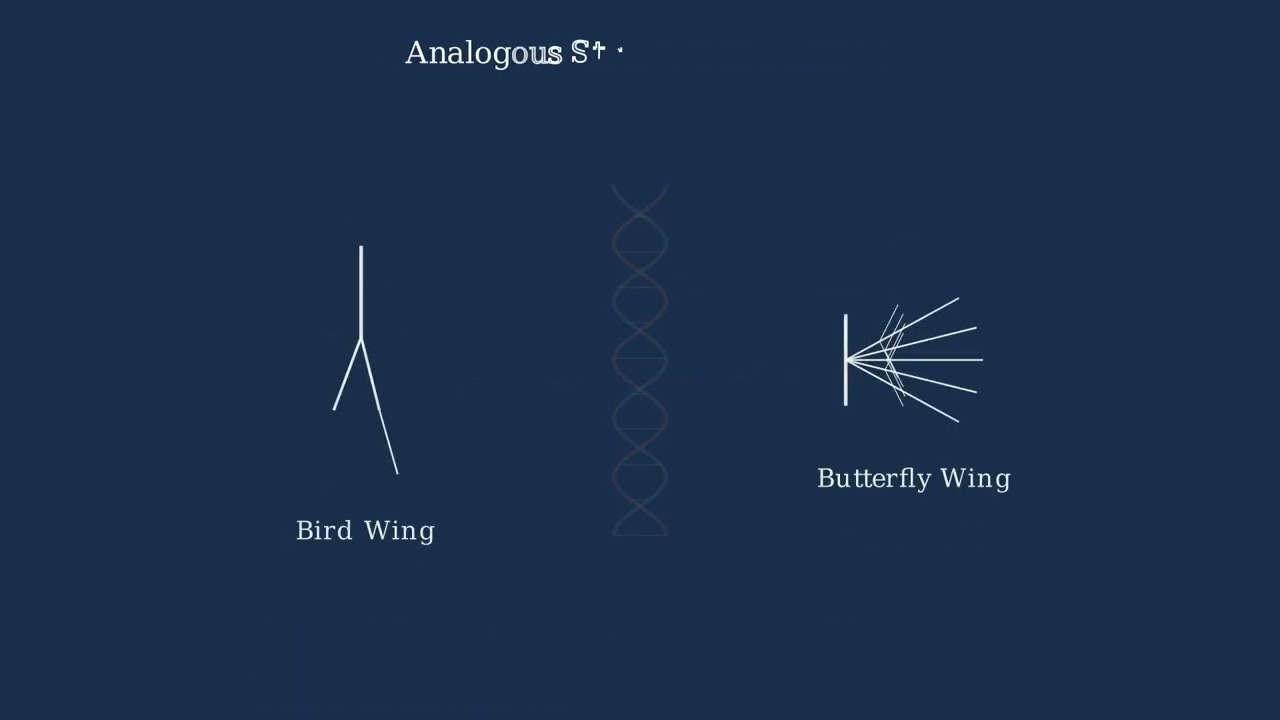
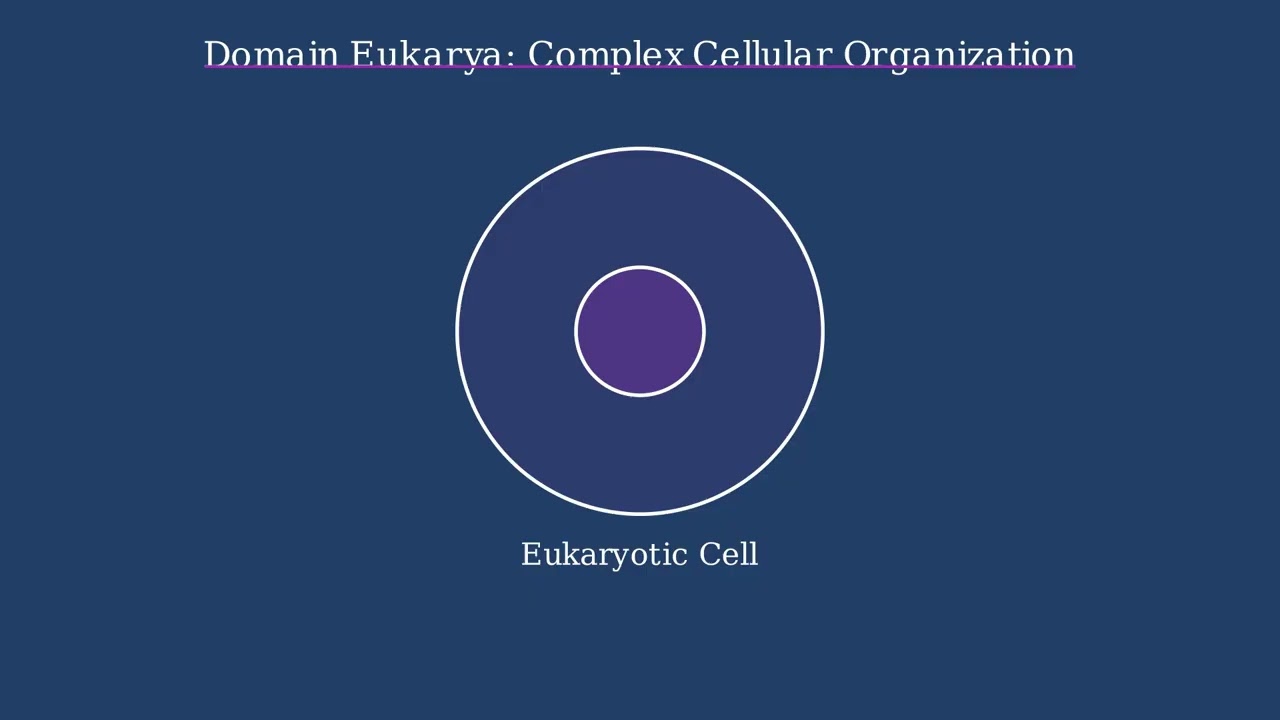
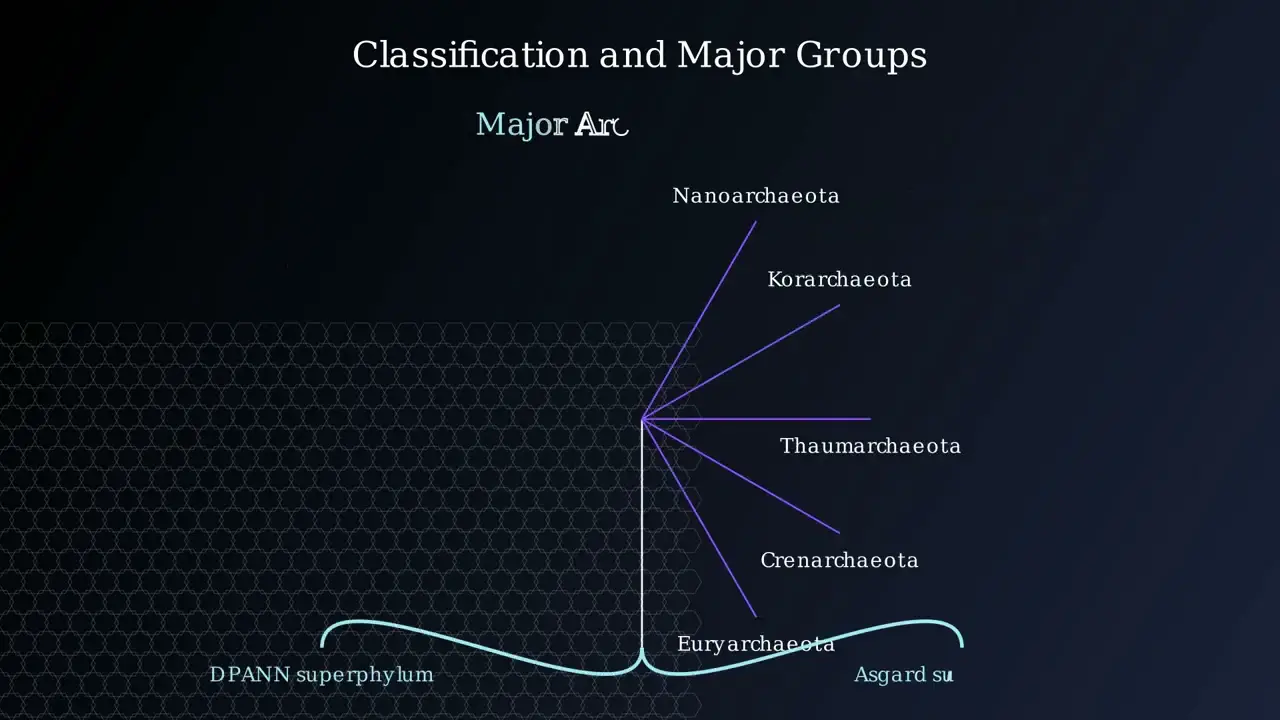
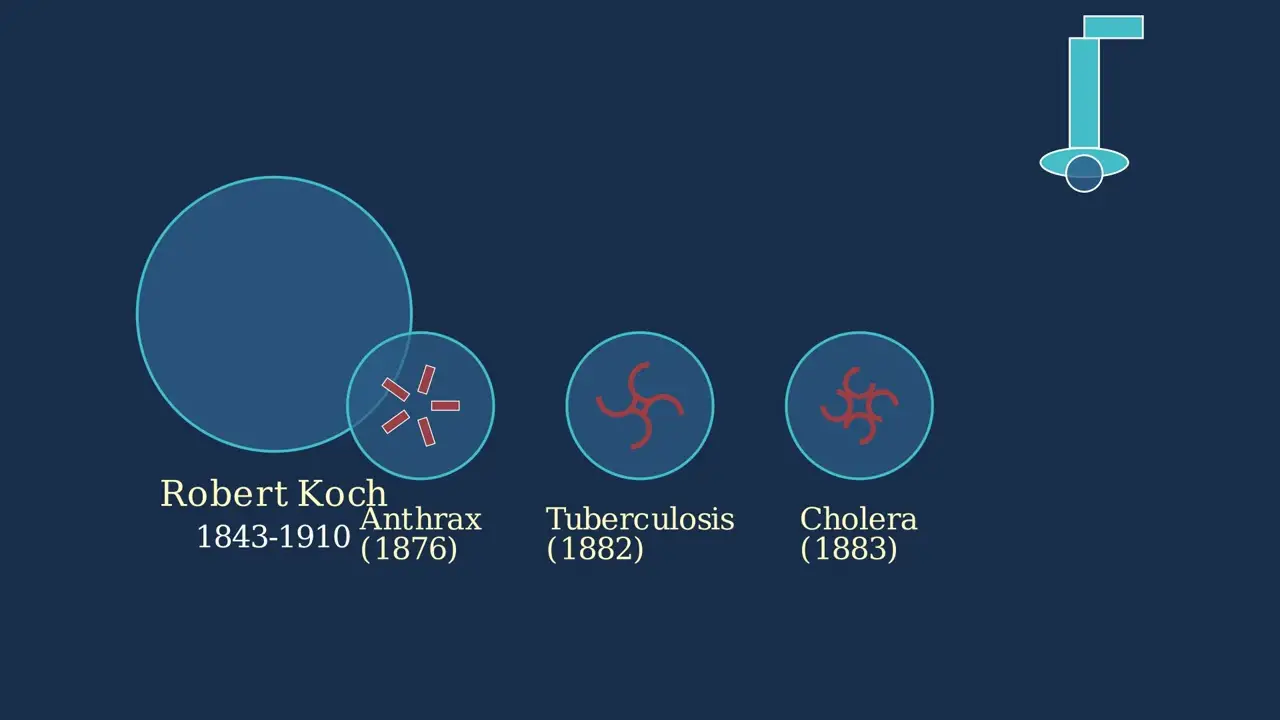
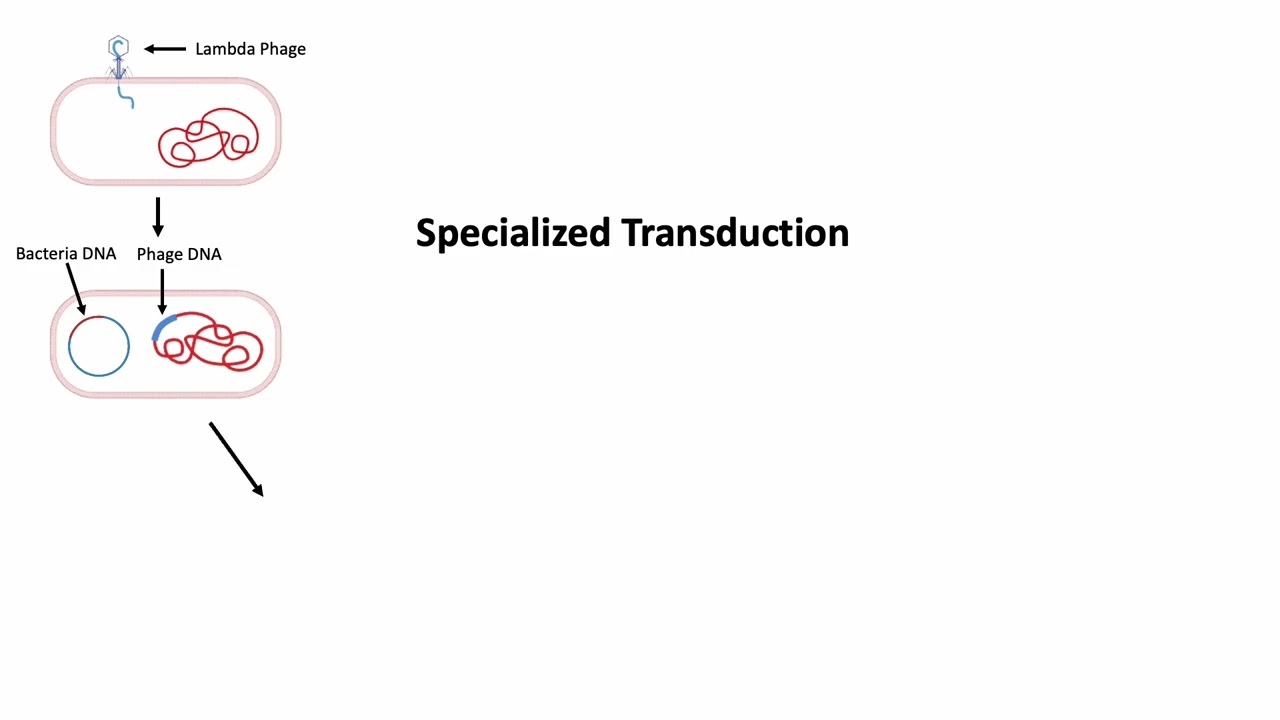
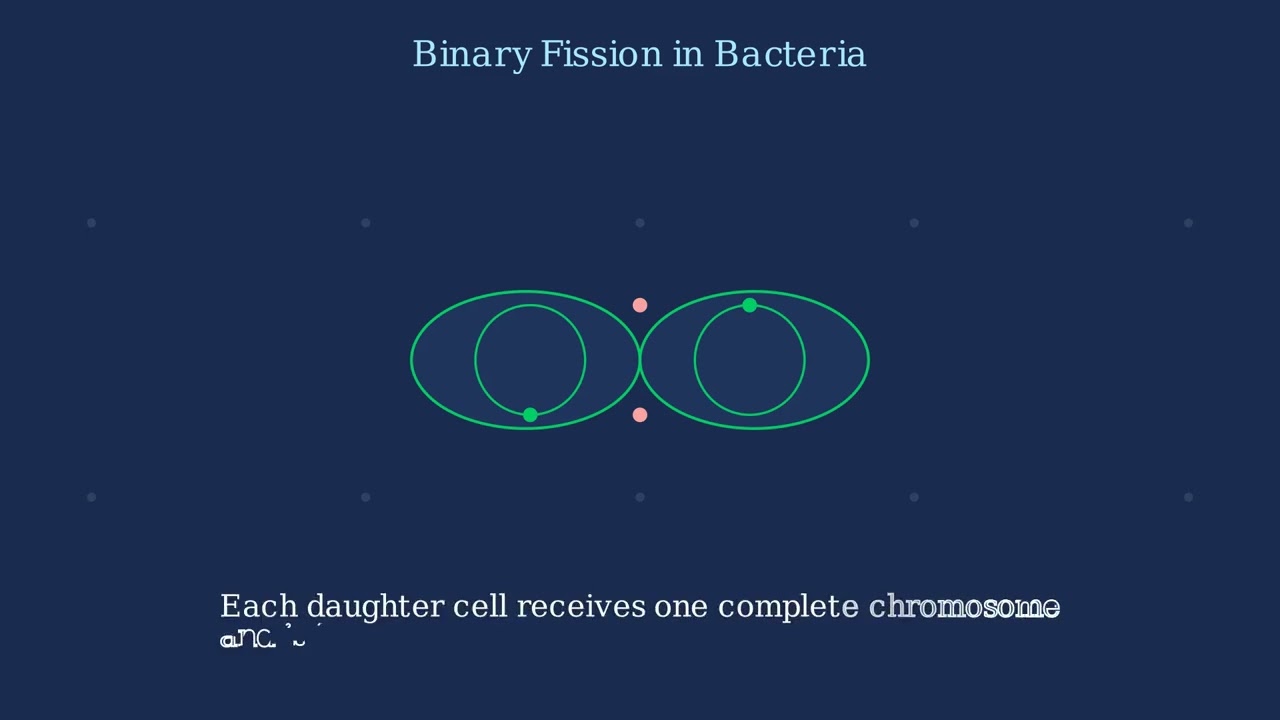
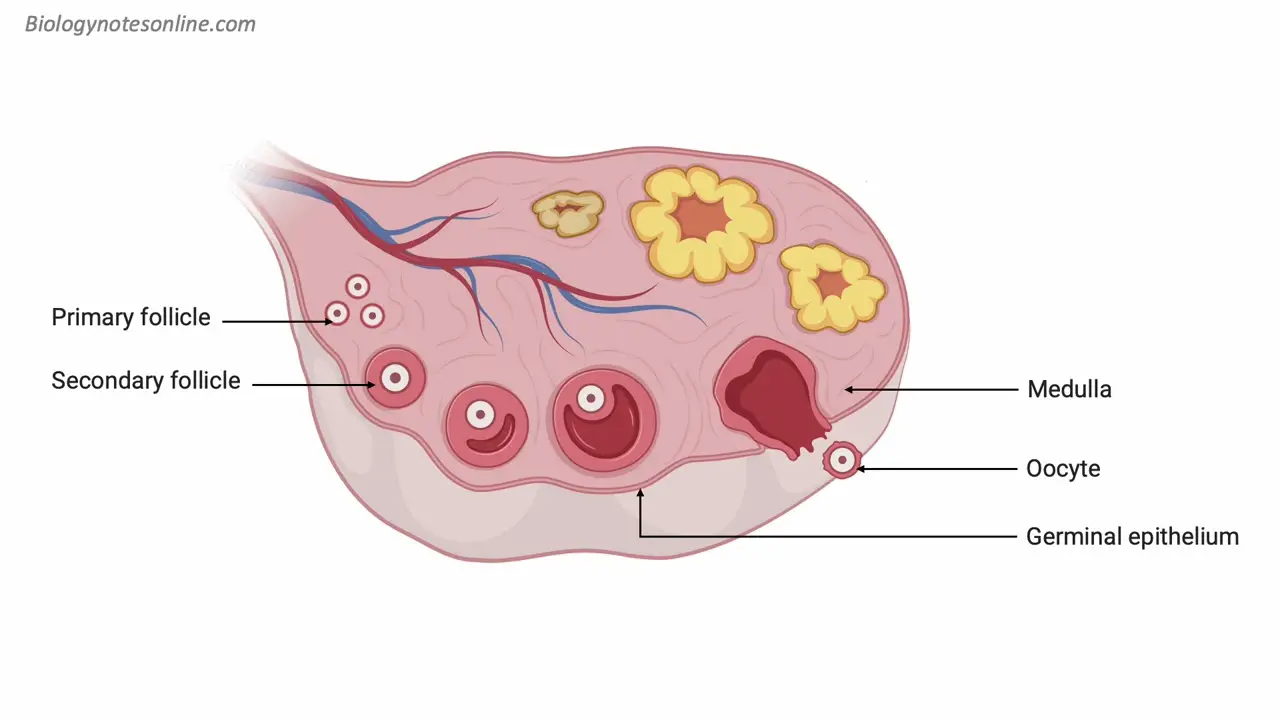
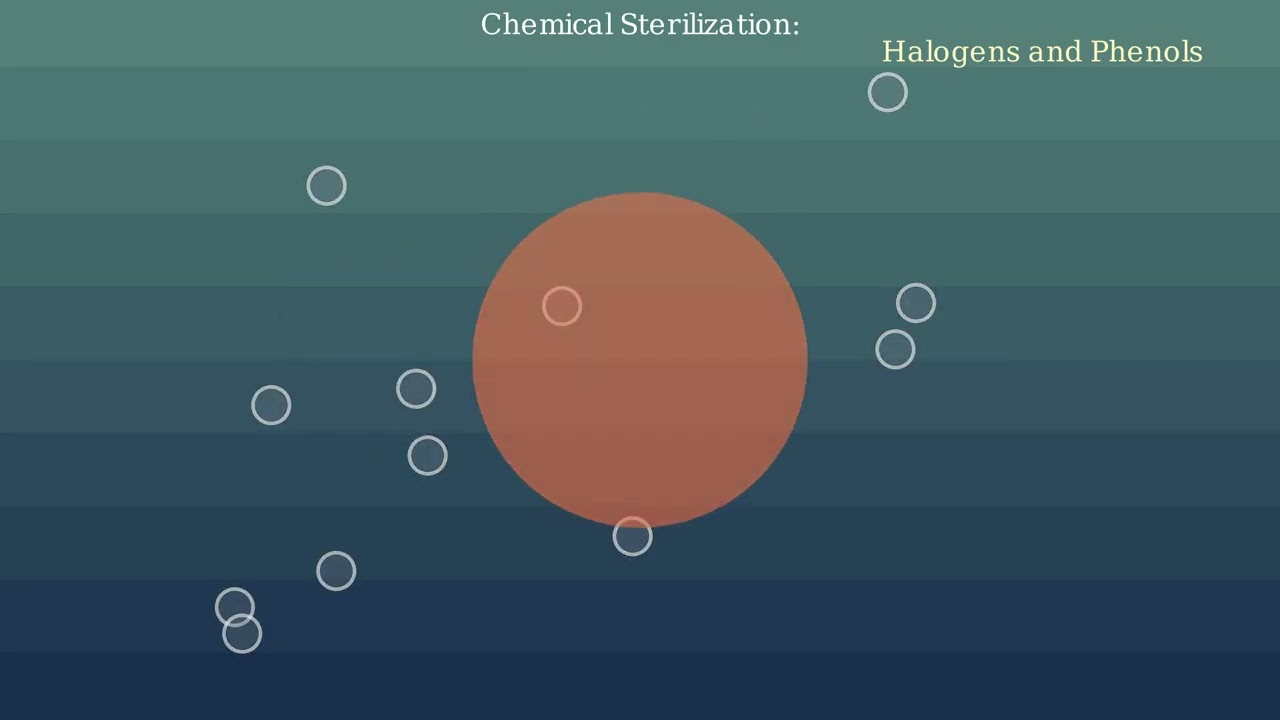