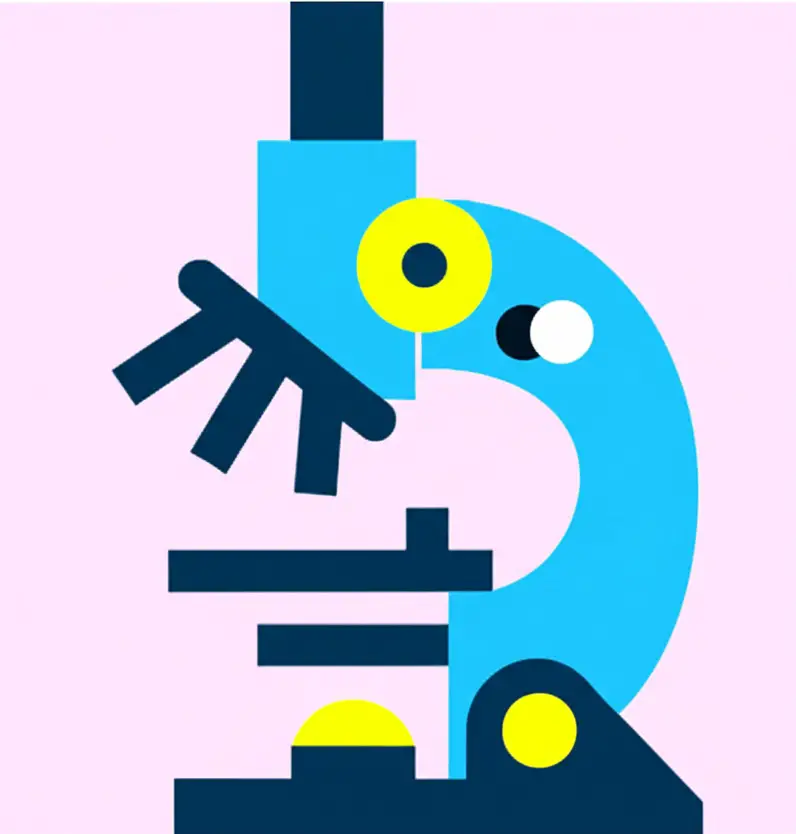
Sourav Pan
Transcript
In controlled experiments, there are three key types of variables.
First, the independent variable is what researchers deliberately change in the experiment.
The dependent variable is what researchers measure to observe the effects of changing the independent variable.
Controlled variables are all other factors that are kept constant to prevent interference with the results.
Let’s look at a concrete example: a plant growth experiment.
In this experiment, the independent variable is the amount of water given to each plant.
The dependent variable is the height of the plants, which we measure to observe the effects of different water amounts.
To ensure valid results, we control all other variables that might affect plant growth.
Let’s look at how these variables work together to establish cause and effect.
The independent variable is what we hypothesize causes an effect.
The dependent variable is what we measure to see if the effect actually occurred.
By changing only the independent variable and measuring the dependent variable, we can establish causation.
This is only possible because we control all other variables that might influence the results.
Let’s summarize the key points about variables in controlled experiments.
Understanding these three variable types is essential for designing and interpreting scientific experiments.
Control and experimental groups form the foundation of controlled experiments.
Let’s examine the essential differences between control groups and experimental groups.
The control group does not receive the experimental treatment. It represents what happens under normal conditions.
In contrast, the experimental group does receive the treatment being tested. This is the only variable that differs between the groups.
Scientists compare results between these groups to identify the effects caused specifically by the treatment.
Why is this comparison so crucial in scientific experiments?
First, it determines if observed effects are truly due to the treatment rather than other factors.
Second, it helps rule out the possibility that changes occurred by chance or due to external variables.
Third, control groups provide a baseline for accurately measuring the magnitude of treatment effects.
Let’s illustrate with a simple example: a plant growth experiment.
In our experiment, we have two identical groups of plants. The control group receives only water.
The experimental group receives the same amount of water plus our treatment: fertilizer.
By comparing the growth in both groups, we can determine that the fertilizer caused an additional sixty percent growth beyond what occurs naturally.
To summarize the importance of control versus experimental groups:
Control groups provide the scientific baseline needed for comparison.
Without control groups, we cannot establish whether the treatment truly caused the observed effects.
The comparison between groups reveals the true magnitude and significance of the treatment’s effect.
Establishing causality is a fundamental goal of controlled experiments.
Let’s first understand the difference between correlation and causality.
Correlation simply means two events occur together or are associated. When one changes, the other tends to change too.
Causality, however, is stronger. It means one event directly causes another. This is what scientific experiments aim to establish.
But establishing causality is complicated by confounding variables.
Confounding variables are unwanted factors that can influence the results of an experiment.
In this diagram, C is a confounding variable that affects both A and B, creating an apparent direct relationship between them.
Without controlling for C, we might incorrectly conclude that A causes B, when in fact both are caused by C.
Controlled experiments help eliminate confounding variables through careful design.
Here are four key methods to eliminate confounding variables:
First, randomization distributes potential confounders equally among groups. Second, control groups allow comparisons where only the independent variable differs. Third, standardized conditions keep all irrelevant factors constant. Fourth, we can measure potential confounders and account for them in analysis.
Let’s explore a concrete example to understand confounding variables better.
Consider ice cream sales and drowning incidents. Data shows they increase together, suggesting a correlation.
But this correlation is misleading. Hot weather is the confounding variable that causes both increased ice cream sales and more swimming, which leads to more drowning incidents.
To establish if there’s a real causal relationship between ice cream and drownings, we would need to control for temperature in our experiment.
By eliminating confounding variables through proper experimental design, we can confidently establish cause and effect relationships.
In summary, controlled experiments establish causality by identifying potential confounding variables and designing controls to eliminate their influence, leading to accurate conclusions about cause and effect relationships.
Reproducibility and standardized conditions form the backbone of scientific credibility.
Reproducibility refers to the ability for different researchers to obtain the same results when repeating an experiment using identical methods.
When multiple labs can reproduce the same findings, it significantly strengthens our confidence in the results.
Reproducibility is crucial because it validates scientific claims, builds trust in the scientific community, helps identify potential methodological flaws, and allows for collective advancement of knowledge.
Standardized conditions form the foundation of reproducibility.
Standardized conditions ensure that all aspects of an experiment remain consistent across different attempts. This includes controlling variables like temperature, timing, measurements, and equipment.
Detailed documentation is essential for reproducibility. Without it, other researchers cannot accurately replicate your experimental conditions.
A thorough documentation checklist includes detailed experimental protocols, equipment specifications, statistical analysis methods, raw data preservation, and notes about any unexpected observations.
The cycle of reproducibility strengthens scientific knowledge. When experiments can be reproduced, findings become more reliable and can be built upon.
In conclusion, reproducibility through standardization is the hallmark of robust scientific methodology.
Study Materials
No study materials available for this video.
Related Videos
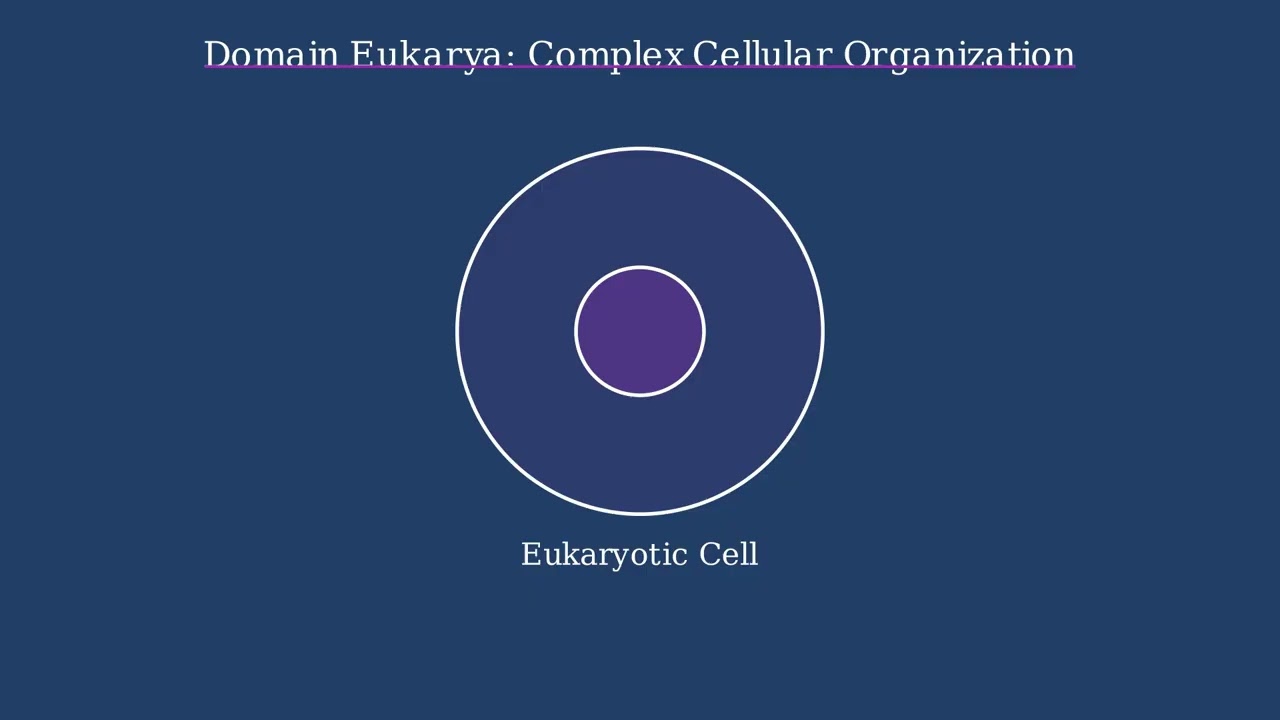
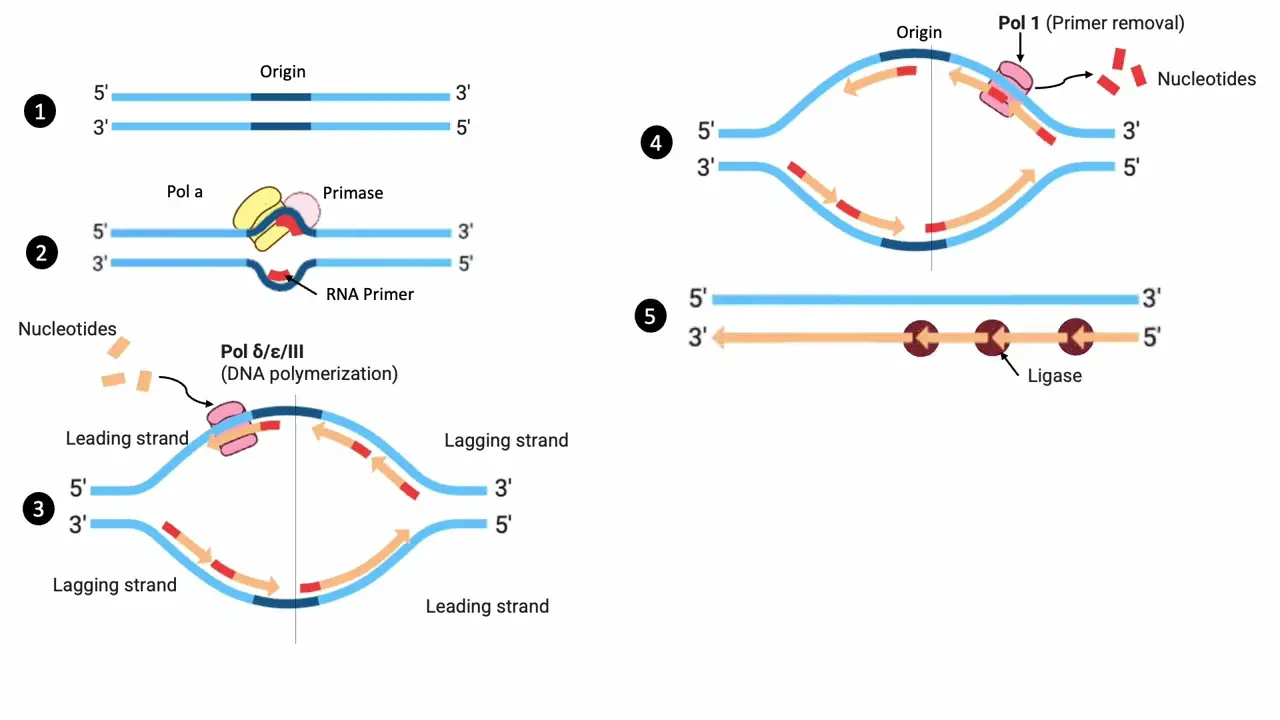
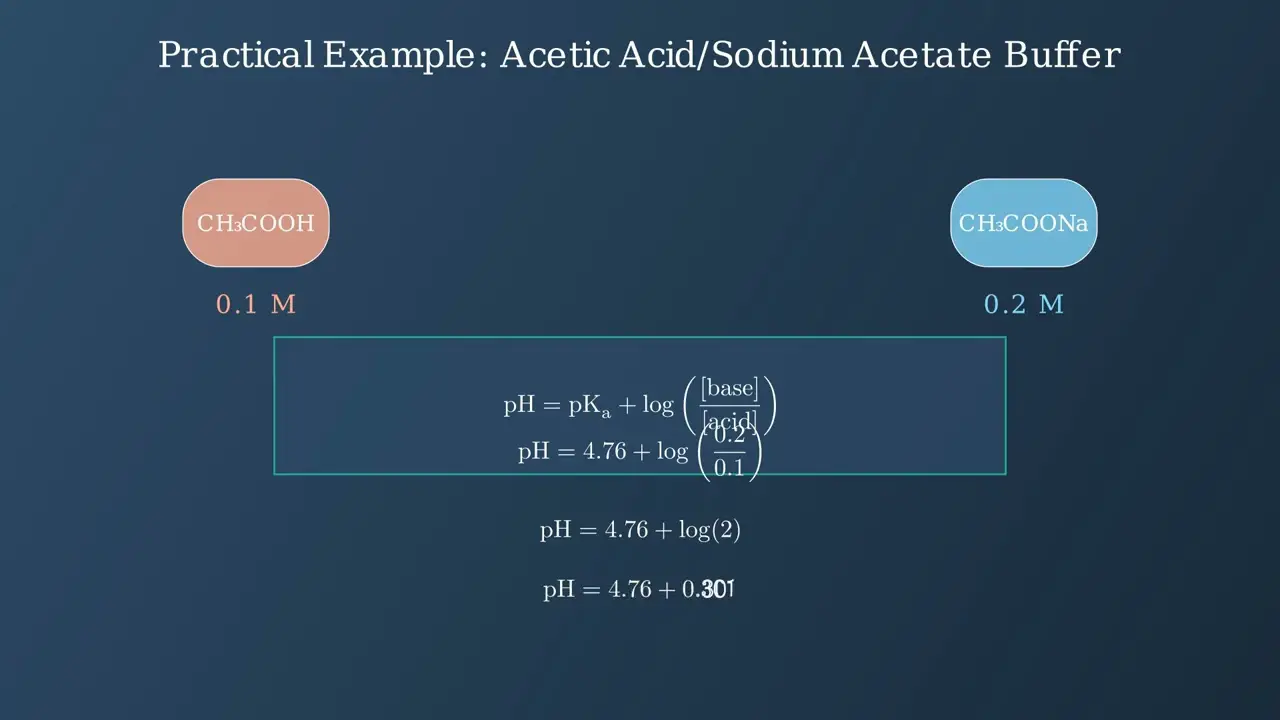
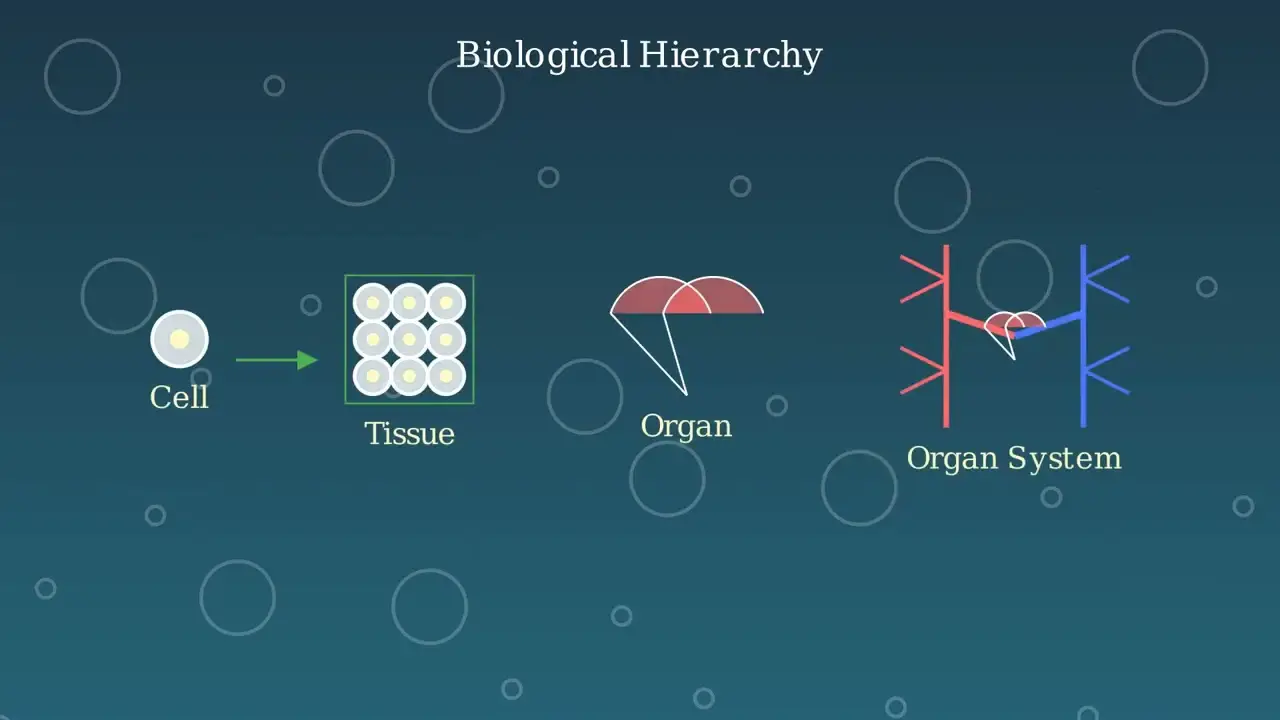
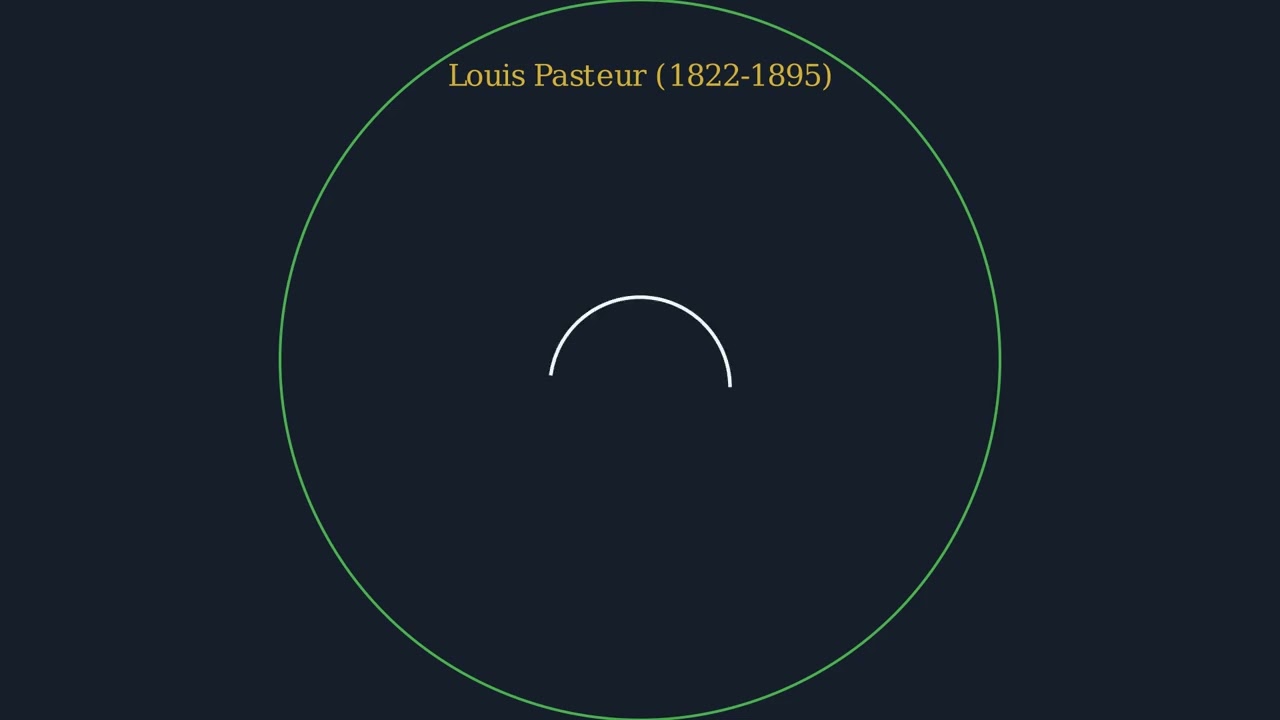
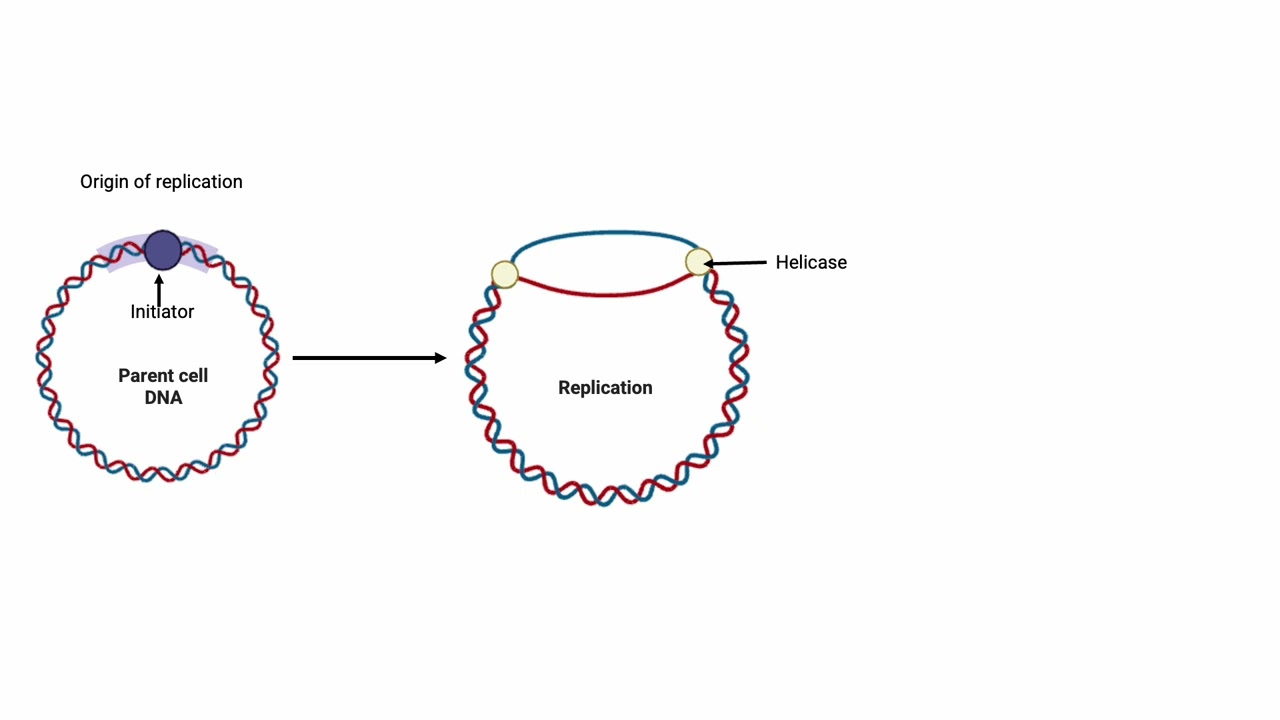
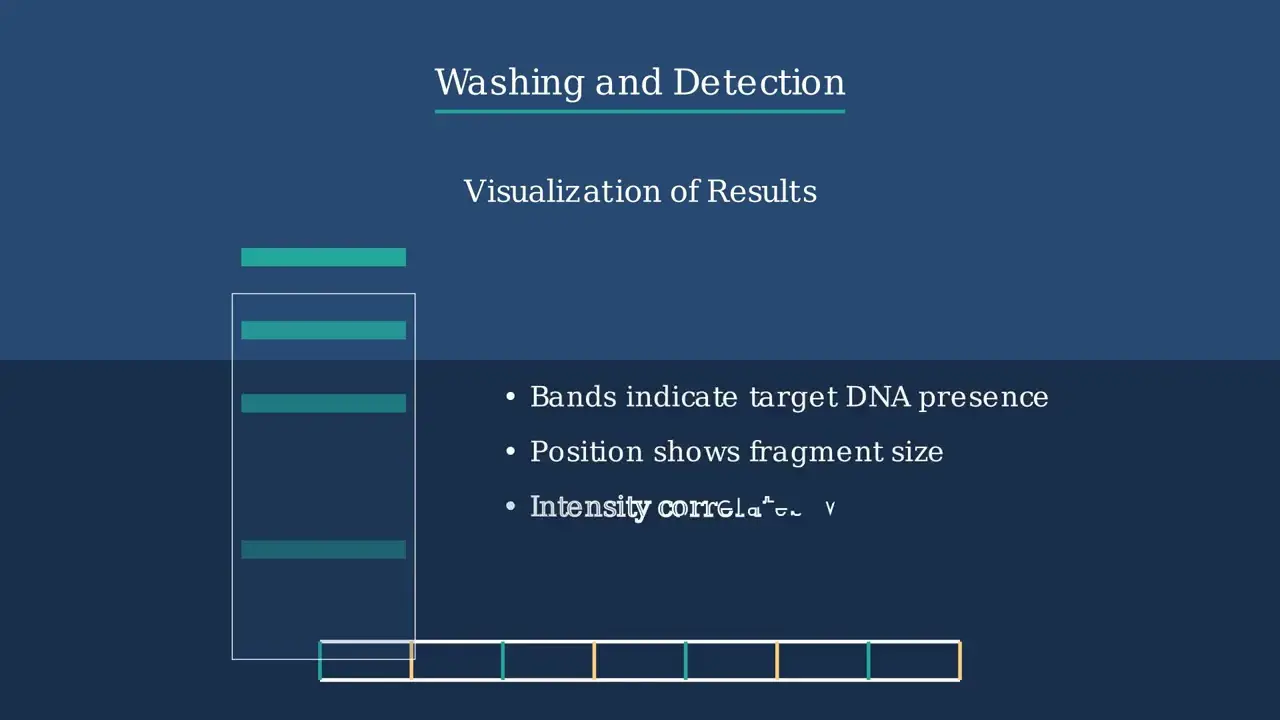
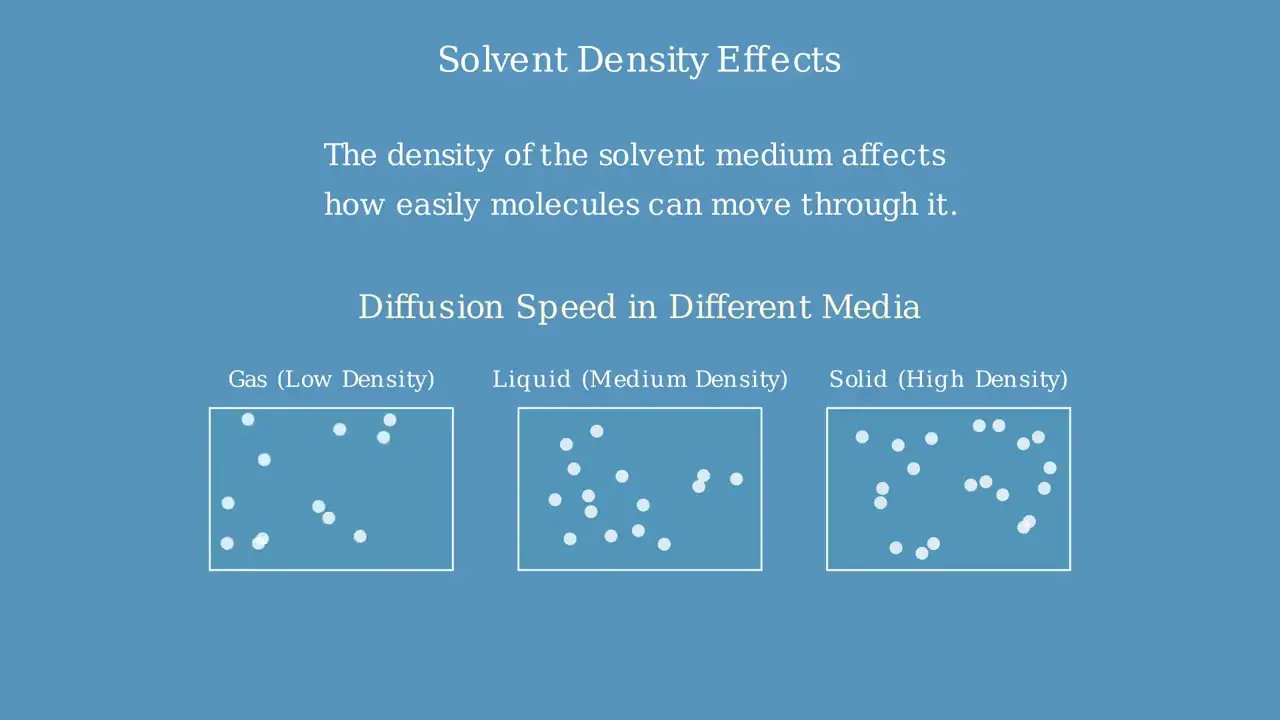
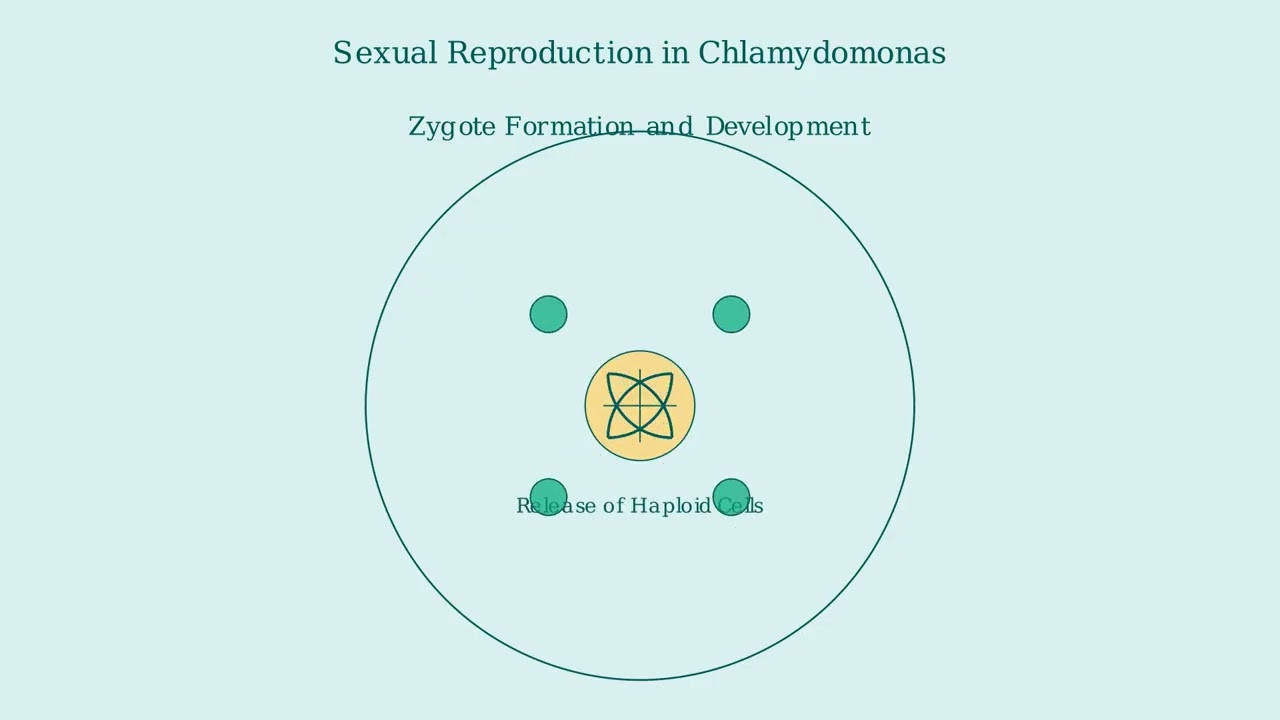
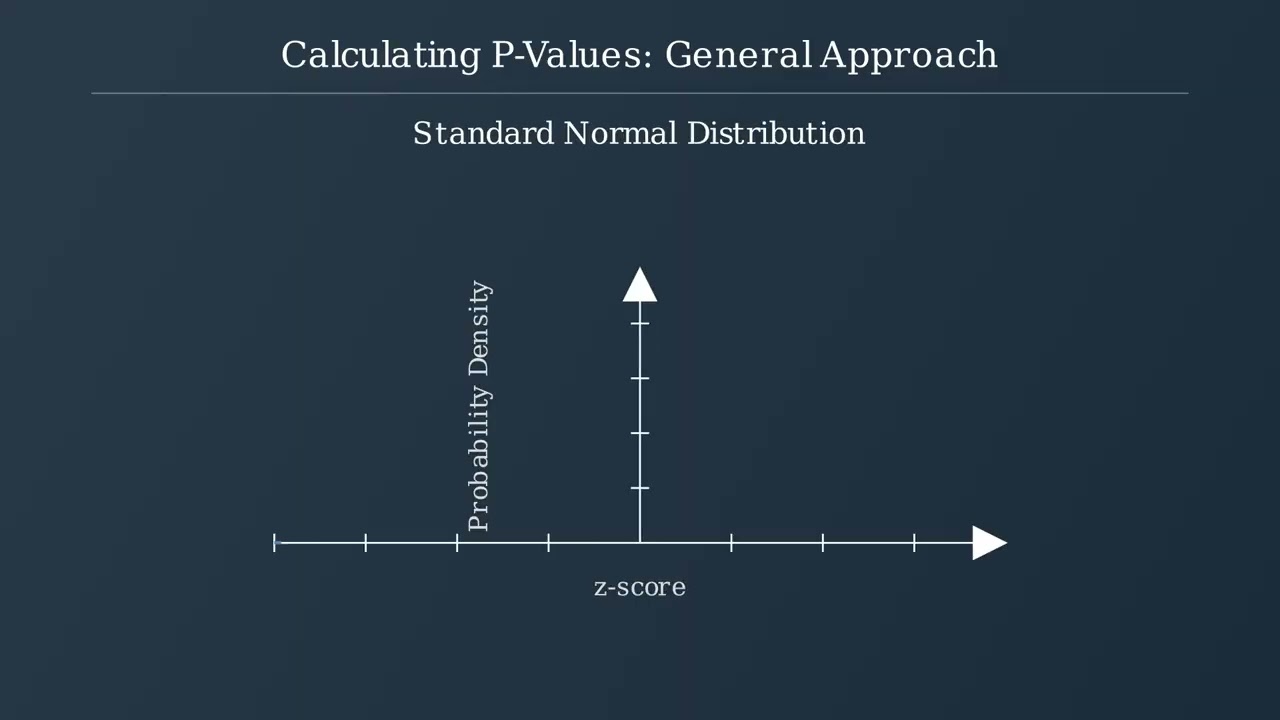